Covid-19 Influence on Mixed Urban Cluster Air Quality: A Case Study from INDIA
Download
Abstract
Introduction: Policy decisions on containment of commercial activity to control the spread of COVID-19 SARS may influence urban air quality.
Methodology: A methodological approach has been proposed to explore the relationship that exists between the policy decisions on six criteria pollutants (PM10, PM2.5, SO2, Ozone, NO2, and CO). This study also explores the knowledge /association that exists between the climatic variables, criteria pollutants, inhalation exposure dose levels and its vulnerable population age group and gender. The methodology explores a mixed urban cluster, Coimbatore, Tamilnadu, INDIA.
Results: From the results, PM10, PM2.5, SO2 and CO has reduced to about 31%, 42%, 26% and 29% during phase-I, whereas NO2 (66%) and Ozone (14%) has increased. It is inferred that the reduced criteria pollutant load (during the phase I, II, and III) gets dispersed taking the advantage of geographical location.
Conclusion: It is also observed that gender has an influence on the inhalation dose due to variability in inflow rate.
Introduction
Pandemic situation has influenced decision makers to formulate policy on contaminant scenarios that may control the spread of COVID-19. The most common containment decision had been lockdown for a known duration with variability of 7 days to 6 months (in certain cases, the single-span extending up to 1 year). This containment decision on urban, and industrial activities may have its footprints on the emission scenarios. The lockdown may have a direct relationship with the criteria pollutant levels in the atmosphere. This demands the research community to explore the influence of containment decisions on a mixed urban cluster (semi-arid zone) in comparison with the pre-lockdown air quality concentration. Further, this variability in criteria pollutants may influence the microclimatic conditions based on the geographical location.
India is facing serious challenges with the deteriorating air quality in metropolitan cities. Air pollution is the fifth most deadly risk factor globally. It has been recognized that breathing in polluted air increases an individual’s chance of developing heart disease (Chronic Obstructive Pulmonary Disease), chronic respiratory diseases, lung infections, and cancer [1]. Until recently, the health effects of Particulate Matter have received prominent attention from the scientific community. US EPA has categorized air pollution into six criteria pollutants viz., Particulate Matter (PM), Ozone, CO, SOx, and NOx based on the sources of emission and the toxicity of the pollutant causing health effects.
In the past decades, due to rapid urbanization and industrialization there has been a plethora number of research works and studies in India. The principal emission of criteria pollutants viz PM10, PM2.5, NO2, SO2, and Ozone is from industries [2,3], vehicular traffic [4-6], domestic fuel burning [7], natural sources (sea salt & soil dust) and unspecified sources of human-induced pollution [8]. The emissions are due to organic and inorganic gaseous PM due to the combustion of fossil fuels (Coal, Petrol, diesel), lubricants, resuspension of mineral dust particles, oil combustion, and unidentified combustion sources from industries [9,10].
On the other hand, COVID-19, SARS (Severe Acute Respiratory Syndrome Coronavirus-2) caused widespread of the virus from the human-to-human transmission, increased contagion, morbidity, and mortality rates, due to which WHO declared COVID-19 as a global pandemic on March 11, 2020. Since the outbreak of the pandemic, billions of people’s lives have been affected also millions of deaths worldwide. Many nations enforced strict lockdown. Numerous studies were carried out in and around the world as shown in Table 1.
Authors | Study Area (City, Country) | Pollutants | Study Period | Key Findings |
[11] | Almaty, Kazakhstan | PM2.5, NO2, SO2, CO, 3, and BTEX | (2020) March 19 to April 14 | Reduction in PM2.5, CO and NO2 of about 21%, 49% and 35% during lockdown period. Increase in O3 of about 15%. |
[12] | New York City, USA | PM2.5, NO2 | (2015-2020) January – May (17 weeks) | Decreases in PM2.5 (36%) and NO2 (51%) concentrations were observed |
[13] | Wuhan, China | PM2.5, PM10, NO2, SO2, CO, O3 | (2020) 1-22 January and 23 January-29 February | During the lockdown period reduction in PM2.5 and NO2 is about 35% and 60%. |
[14] | North China Plain | PM2.5, PM10, NO2, SO2, CO & O3 | (2020) January 23 - March 15 | Reduction PM2.5 of about17.7% during lockdown period. |
[15] | Indian Cities Twenty-Two | PM10, PM2.5, CO, NO2, O3 and SO2 | (2017-2020) 16 March – 14 April | Reduction in PM2.5-43%, PM10-31%, CO-10%, and NO2-18% during lockdown in comparison with previous years. |
[16] | New Delhi, Chennai, Kolkata, Mumbai and Hyderabad | PM2.5 | (2020)(1st March–24th March), (25th March–31st May) and (1st June–31st August) | During lockdown period decrease in PM2.5 of about 21.3%, 48.5%, 63.4%, 56.4%, and 23.8% in New Delhi, Chennai, Kolkata, Mumbai and Hyderabad. |
[17] | Delhi, Mumbai, Chennai, Kolkata and Bangalore | PM10, PM2.5, NO2, O3 and CO | (2019 and 2020) March–April | Except ozone there was statistically significant reduction in all the criteria pollutants. During lockdown reduction in PM2.5, PM10, NO2 and CO are about 41%, 52%, 51% and 28% at Delhi respectively. |
[18] | Delhi | PM10, PM2.5, CO, NO2, O3,, SO2 and NH3 | (2020) 2 March –21 March & 25 March- 14 April | The highest reduction in concentrations of PM10 (60%), NO2 (53%), PM2.5 (39%), and CO levels (30%) were observed during the lockdown period. |
[19] | Delhi, Kolkata, Mumbai and Hyderabad | PM2.5, PM10, NO, NO2, NOX, CO, SO2, NH3, O3, BTX, and AQI | Jan 2018 to July 2020 | A significant decline in PM2.5 of 43.7% & 49.8% during lockdown period in Delhi and Kolkata. NO2 has reduced to about 55% in Delhi during lockdown period. |
[20] | India | PM10, PM2.5, CO, NO2, O3, SO2 and NH3 | (2020) February 25 - March 20 & March 25 - April 25. | A reduction in tropospheric levels of NO2, O3 and LST was observed post- lockdown period. Improvement in AQI in India |
[21] | Ankleshwar and Vapi of Western India | PM10, PM2.5, CO, NO2 and O3 | (2020) 25 March – 31 May | PM10, PM2.5, CO and SO2 has rapidly reduced during the lockdown period. Drastic reduction in NO2 and increase in O3. |
[22] | Beijing | NO2 and PM2.5 | (2015 – 2019) January to June | The results revealed that lockdown measures caused large reductions while meteorology offset a large fraction of the decrease in surface concentrations. Decline in NO2 concentration to about 42% at the start of the lockdown. |
[23] | European Regions -Northern Italy | Wind climatology, Particulate Matter and Ozone | (2018,2019) February to April (2020). | Reduction in dispersion of particulate pollutant based on the low wind speed (atmospheric stability). Polluted cities with unstable atmosphere and high wind speed can decrease air pollution having viral agents commingled with particulate matter, and alleviate the lethality and diffusion of the Coronavirus. |
In India, the first complete lockdown has been started on March 24th, 2020 - Janata Curfew, which extended till May 31st, 2020. During this period strict restrictions for people movement, transportation sector, industrial activities, domestic and international flights, workplaces, and day-to-day activities. States and Union territories under the Government of India’s jurisdiction followed the policy decision of lockdown. The above policy decision has a strong footprint on the air pollution emission scenarios.
Several studies have been carried out in India during the COVID-19 pandemic. Reduction in CO2 at Kolkata and West Bengal to about 30-40% has been observed [24]. It is inferred that PM10 reduction of about 52%, NO2 (51%) and CO (28%) in major metropolitan cities viz Delhi, Mumbai, Chennai, Kolkata and Bangalore [17]. Delhi has a major decline in the concentration of the criteria pollutants viz PM10, PM2.5, CO, NO2 during the lockdown in comparison before lockdown [18]. Reduction in PM2.5 has been observed in Delhi (53%), Chennai (39%), Kolkata (36%), Hyderabad (54%) during the lockdown in comparison with the previous four years [15]. The relationship between environmental and demographic variables has been established with COVID-19 cases and air quality [24]. Similarly, the relationship between infected people and environmental, demographic, and geographic factors has influenced the spread of novel COVID-19 [25]. From the above, it is observed that PM2.5, PM10 and population density has a positive association with mortality and morbidity. Further, the spread duration of COVID-19 has a high correlation with population density and absolute humidity, whereas the decay duration of COVID-19 is highly correlated with the population density, absolute humidity, and maximum temperature [26]. In addition, the relationship between atmospheric and environmental factors has been statistically established with the number of infected people [27]. In general reduction in anthropogenic activities due to COVID-19 has positive impacts (air quality, water quality, noise intensity, municipal waste generation, and improved forest ecosystem) and negative impacts(increased ozone levels, biomedical, plastic, and supply chain wastes, poaching of wildlife and deforestation and illegal extraction of resources) on environmental components [28]. Similarly, a longer period of lockdown has a negative impact on economic growth [29].
Most of the above-mentioned research works focus on metropolitan cities considering the spread of urban population settlement, and the probability of emission sources. However, in the case of a developing country like INDIA, geography and population migration supports the transformation of small urban clusters into future major urban dwelling units. One such transforming mixed (urban and industrial) cluster is Coimbatore (a city in the administration of Tamilnadu state, INDIA), where this study is conducted to understand the impact of policy decisions made during pandemic on the urban air quality. Coimbatore comprises more than 25,000 industries in the city this includes (small, medium, and large textile, electro-plating, and foundries). The total number of registered vehicles in Coimbatore city is about million. Urban agglomeration has a strong influence on the increasing PM levels in the city. The existing and prevailing weather pattern is not in the general notion with particular dilution and dispersion. The above studies have proved that temperature, wind speed, and wind direction have a positive correlation with pollution (PM2.5) concentration in the city vicinity [30]. Respirable 2.05 Suspended Particulate Matter (RSPM) has a significant positive correlation with the sources emitted from urban and industrial areas [31]. The air sampling carried at Small Industries Development Corporation (SIDCO), Lakshmi mills, Kuniyamuthur, Kavundampalayam, and 100 feet has PM2.5 concentration between 27.85 and 165.75 µg/m3 that is exceeding Central Pollution Control Board (CPCB) standards in most of the sampling locations. It is inferred that in the urban and suburban areas of Coimbatore city the RSPM is exceeding the CPCB standards [30]. This may be due to Haphazard urbanization and unprecedented vehicular growth. The above demands more robust and vigorous studies related to air quality are required for the developing urban region. The above research findings highlight that the air quality is getting polluted which may be due to urban activities / industrial / traffic emission scenarios.
From the above studies, it is inferred that the containment decisions have influenced the air quality pollutant levels. This research is structured to address major research gaps, i) the extend of contaminant decision on criteria pollutant levels (PM10, PM2.5, SO2, Ozone, NO2, and CO) in a mixed urban cluster (a cluster that is in the transformation phase of the metropolitan city), ii) the influence on contaminant decision on the microclimatic condition (Temperature, Relative Humidity, and Rainfall), and iii) the influence on inhalation exposure dose with respect to population age group, and gender.
The rest of this research is organized to explicitly showcase the methodology/framework that is been used to explore the database of the chosen criteria pollutants and the climatic database. To visualize the mixed urban cluster, the database on Coimbatore, Tamilnadu, India, is summarized. The proposed methodology is allowed to explore the database, and the recovered relationship/ knowledge is discussed. A conclusion illustrates the summary of the research, with major inferences and probable further research that may be explored.
Materials and Methods
A methodological framework is proposed to address the intended research gaps with three major components,
a) Database analysis to understand geographical location and mixed urban cluster, b) Data pre-processing and analysis of criteria pollutants, c) Data pre-processing and analysis of Climatic variables, and d) Inhalation exposure dose assessment.
Geographical Location & Mixed Urban Cluster
Coimbatore (11.0168°N, 76.9558°E.) (Figure 1) is located on the west corner of the south Indian state of Tamil Nadu, surrounded by the Western Ghats on all sides.
Figure 1. Coimbatore Location Map.
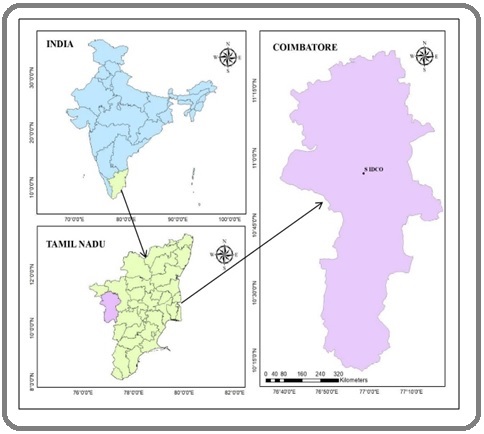
The average elevation of Coimbatore above the MSL is ~398 m. The geographic position of the city, in the immediate east of the pass, the breach of Palghat, through the Western Ghats offers direct access to West Coast traders has fostered its commercial prosperity.
The city has an overall population of ~1.05 million with a population density of about 601 persons per square kilometer. It is the Manchester of South India with the second-highest population in Tamil Nadu. The total number of registered vehicles in Coimbatore city is about 2.05 million. Figure 2, represents the land use and land cover map of Coimbatore city.
Figure 2. Land use and Land Cover map of Coimbatore City.
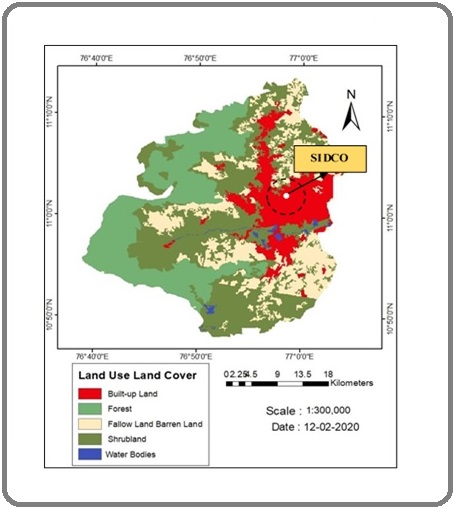
The industrial sector of the district accounts for approximately 7.84% of the land in the urban city of Coimbatore. Coimbatore’s industries produce a variety of engineering products ranging from small plastic items to major textile machines.
Contaminant Decisions
Figure 3, summarizes the contaminant decisions that are taken and implemented by the decision-makers to control the spread of COVID-19.
Figure 3. COVID-19 Timeline Frame of Tamil Nadu (Lockdown and Relaxations).
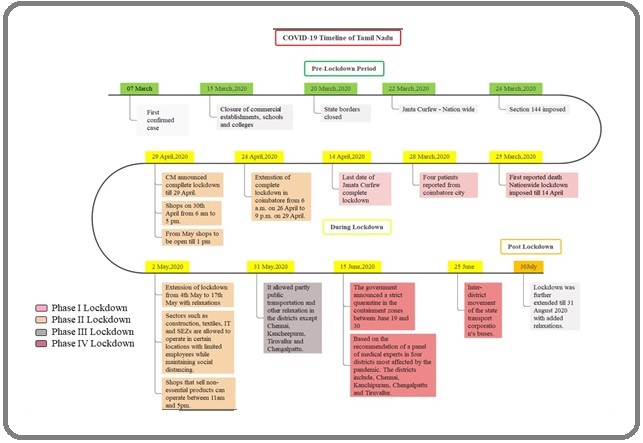
From Figure 3, it may be observed that during phase I of lockdown complete restrictions for all the activities (urban, and industrial) including restrictions for peoples’ movement. During phase II of lockdown, commercial outlets (shops) were allowed to open with a constraint on operation time. Phase II also allowed industries to operate with 50% manpower capacity. Phase III lockdown relaxed furthermore with the decision on permitting public transportation operation to facilitate the movement of people and raw materials. Phase IV of lockdown is a kind of regular operation scenario with few restrictions.
Data Source
This research handles two kinds of data, i) data about six criteria pollutants (PM10, PM2.5, SO2, Ozone, NO2, and CO), and ii) database of climatic variables (Temperature, Relative Humidity, and Rainfall). The databases of criteria pollutants are taken from a monitoring station located at SIDCO, Kurichi (Figure 1) maintained by Central Pollution Control Board (CPCB, Continuous Ambient Air Quality Monitoring (CAAQM)). The 24-hours concentration from January 01, 2020, to October 31, 2020, of six criteria pollutants PM10, PM2.5, NO2, SO2, CO, and Ozone has been obtained from the CPCB online portal. To understand the behaviour of climatic variables in various phases of lockdown, the historical database from January 2019 to May 2021 is utilized from IMD (Indian Metrological Department) monitoring station.
Data processing and analysis
The data obtained from CPCB & IMD was further explored for its statistical characteristics a) Arithmetic Mean b) Standard Deviation c) Maximum value and d) Minimum value which is represented in Table 2 and 3. The database is subjected to pre-processing analysis to identify the noise and missing values in the monitored time series. From the raw database, it is observed that the data gap is less than 1% of the total data length. The identified data gaps are replaced using the weighted average method.
W = ∑ni=1 wi .xi / ∑ni=1 wi (1)
Where ‘W’ is the weighted average; ‘n’ is the number of terms to be averaged; wi is the weights applied to x values and Xi is the data values to be averaged.
Correlation analysis [32] is performed to explore the relationship/association that exists between the variables (criteria pollutant, climatic variables, criteria pollutants, and climatic variables). The historical database has been prepared and the same is subjected to correlation analysis considering dependent variables and other independent variables.
Correlation Coefficient = ∑ (x-x ̅)(y-y ̅) )/(n-1) sxsy (2)
where, Sx and Sy represent the sample standard deviations of the x and y data values, respectively. The correlation coefficient takes on values between 1 and -1, and if the correlation coefficient carries a positive sign, it indicates that the dependent variable and the independent variable considered for assessing the correlation coefficient are positively correlated i.e., there exists a relationship that variables considered are direct, proportional. On the other hand, if the correlation coefficient carries a negative sign, it indicates that the variables are negatively correlated i.e., variables are inversely proportional to each other.
Dose estimation
Inhalation exposure dose can be estimated by knowing the contaminant(air) concentration (mg/m3), air intake rate (m3/day), exposure factor (unitless) and body weight (kg). The following equation (3) is used for estimating the inhalation exposure dose [33].
Inhalation exposure dose (D) = (C x IR x EF) / BW (3)
This equation is used in the recent study [34]. The average concentration of PM2.5 during different phases of lockdown were used for estimating the inhalation exposure dose. Air intake rates suggested Exposure factors handbook of United States Environmental Protection Agency (1997) were utilized in this study. Air intake rate for girl 12-14 years is 12 m3/day, boy 12-14 years 15 m3/day, female 19 – 65+ years is 11.3 m3/day and male 19 – 65+ years is 15.2 m3/day.
Results
Criteria pollutant
The monthly average concentration of the criteria pollutants PM10, PM2.5, NO2, SO2, CO, and Ozone reported in CPCB monitoring station in the time of the Pre - Lockdown Period, During-Lockdown Period and Post-Lockdown period is shown in Figure 4. It was observed that during the lockdown period PM10, PM2.5, NO2, SO2, and Ozone concentrations seem to be minimum in comparison with a pre-lockdown period in Coimbatore city. During phase I of lockdown there was a sudden decrease in PM10 (31%), PM2.5 (42%), SO2 (26%) & CO (29%) concentration, and an increase in NO2 (66%) and Ozone (14%) concentration respectively in comparison with the pre-lockdown period. The reduction in PM10 and PM2.5 levels may be largely attributed to reduced emissions from various sources, especially to highlight emission from the transportation sector may be reduced due to restricted mobility to workplaces, colleges, and schools within the administrative boundary of Coimbatore. From Table 2, it is also observed that there is a reduction in SO2 this may be due to reduced commercial activities and in and around the city vicinity.
Pollutants | Pre-Lockdown | During-Lockdown | Post-Lockdown | |||
Phase-I | Phase-II | Phase-III | Phase-IV | |||
Jan 01 - Mar 24 | Mar 25- Apr 19 | Apr 20 - May 03 | May 04 - May 30 | May 31 - Jun 30 | Jun 31 - Oct 31 | |
AM ± SD | AM ± SD | AM ± SD | AM ± SD | AM ± SD | AM ± SD | |
[Max-Min] | [Max-Min] | [Max-Min] | [Max-Min] | [Max-Min] | [Max-Min] | |
PM2.5 (ug/m3) | 37.8 ± 7.7 | 25.9 ± 6.2 | 20.3 ± 5.6 | 22.6 ± 6.8 | 14.8 ± 3.5 | 21.3 ± 9.2 |
58.25 - 22.25 | 34.87 - 10.43 | 25.14 - 2.82 | 32.42 - 4.29 | 24.03 - 8.12 | 53.63 - 3.27 | |
PM10 (ug/m3) | 51 ± 12.9 | 29.79 ± 14.8 | 25.2 ± 3.4 | 30.6 ± 8.8 | 21.3 ± 5.1 | 31.0 ± 11.8 |
108.54 - 1.6 | 95.42 - 11.77 | 31.17 - 20.58 | 45.97 -7.41 | 36.47 - 12.71 | 81.72 - 4.85 | |
NO2 (ug/m ) | 39.7 ± 17.1 | 66.0 ±6.9 | 53 ± 17.7 | 34.5 ± 15.1 | 43.01 ± 5.9 | 21.5 ± 10.6 |
83.74 - 2.76 | 78 - 54.06 | 78.18 - 27.41 | 54.87 -7.41 | 57.65 - 33.43 | 52.47 -3.82 | |
SO2 (ug/m ) | 9 ± 2.4 | 6.636 ± 0.8 | 6.5 ± 0.4 | 6.1 ± 0.4 | 5.9 ± 0.4 | 5.8 ± 1.6 |
14.93 - 1.44 | 8.83 -5.77 | 7.26 - 5.94 | 7.23 - 5.36 | 6.81 - 5.32 | 7.56 - 0.84 | |
CO (mg/m3) | 0.51 ± 0.3 | 0.6 ± 0.1 | 0.9 ± 0.4 | 0.9 ± 0.4 | 0.7 ± 0.2 | 0.5 ± 0.2 |
1.04 - 0.09 | 0.66 - 0.52 | 1.53 -0.68 | 1.53 - 0.07 | 1.06 - 0.42 | 1.21 - 0.02 | |
Ozone (ug/m3) | 44.9 ± 12.2 | 32 ± 10.1 | 20.8 ± 3.3 | 20 ± 4.0 | 13.3 ± 2.9 | 9.6 ± 3.7 |
77.69 - 18.54 | 49.71 - 19.47 | 27.97 - 16.94 | 27.45 - 13.76 | 20.54 - 10.12 | 23.44 - 4.44 |
However, it is inferred that there is an increase in NO2 levels this may be from industrial plumes that could with the limited human resource as per the policy decision [35]. The pattern of increase in ozone levels may be due to increased concentrations at the surface levels during the phase I lockdown. Further, during the phase II lockdown, it may be observed a pattern of decrease in the concentration PM10 (15%), PM2.5 (22%) [36], NO2 (20%) [37], SO2 (1.5%) & ozone (35%) and increase in CO (63%) concentration Table 2. The decrease in concentration levels during the phase II lockdown with phase I lockdown is comparatively less when compared to the pre-lockdown period. Because during phase I lockdown from March 25, 2020, to April 19, 2020, there were strict restrictions for all the activities like transportation sector, industrial sector, and local movements. Thus, during phase II lockdown, shops were opened during restricted timings which led to the local peoples’ movement which contributed to mild vehicular emission thus less emission of concentration was observed. During phase III & IV lock down the pollutant concentrations started to increase in PM10 (15%) and PM2.5 (22%) & decrease in NO2 (24%), SO2 (3.5%), CO (29%) & ozone (33%). The increase in PM10 and PM2.5 concentration is because the state government announced the extension of lockdown with certain relaxations like the operation of construction sites, Textile Industry, Special Economic Zones, and IT sector with limited manpower maintaining social distance in Coimbatore city. However, there was a hike in concentrations during the post-lockdown period. Increase in PM10 (44%) and PM2.5 (45%), decrease in NO2 (50%), SO2 (2.5%), CO (28%) and Ozone (28%) concentration was observed. The increase in PM10 and PM2.5 is due to the emission from the industrial plumes as industries were allowed to operate. The CAAQM station is located in SIDCO, Coimbatore which is an industrial area with a cogent number of electroplating, textile, and motor industries. Thus, these industries may influence the increase in particulate pollutants.
The correlation analysis was performed for the criteria pollutants PM10 (ug/m3), PM2.5 (ug/m3), NO2 (ug/m3), SO2 (ug/m3), CO (mg/m3), and Ozone (ug/m3) during the Pre-Lockdown Period, During-Lockdown Period and Post-Lockdown period. Daily data of January 1, 2020, to October 31, 2020, are taken for the study, and the correlation results are represented in Table 3. R ≥0.5, 0.25≤R<0.5, and 0 Table 3. Correlation Coefficients of the Criteria Pollutants. Similarly, -0.25 The percentage contribution of PM10 and PM2.5 during the lockdown has been reduced in comparison with pre-lockdown and post-lockdown as shown in Figure 5. Similarly, the percentage of NO2 has gradually increased during the lockdown period in comparison with the pre-lockdown and post-lockdown period [38,39]. Post-lockdown similar kind of mixed results (PM10, PM2.5, NO2, SO2, CO, and Ozone) has been observed in other megacities in India [40]. On the other hand, the above reduction of PM levels as a result of a policy decision of lockdown has reduced the risk of particulate matter emitted which causes serious health effects such as Chronic Obstructive Pulmonary Disease (COPD), Cardiovascular disease, Ischemic Heart Disease and Respiratory diseases [41-43]. However, it is observed that all the criteria pollutant levels reported during Phase I and Phase II lockdown are within the permissible limits (CPCB standards) viz PM2.5, NO2, SO2, CO, and Ozone, except PM10 which has exceeded during the pre-lockdown period. This shows that the pollutant concentrations are well within the limits, but due to the rapid increase in population and vehicular count, it may be inferred that the pollutant concentration might cross the permissible limits in the future. Further, all the criteria pollutants are at the verge of the permissible limit. NO2 concentrations have increased during the lockdown period in comparison with pre-lockdown and post-lockdown which is represented in Figure 4. Figure 4. Graphical Representation of PM10, PM2.5, NO2, SO2, CO and Ozone During Pre-Lockdown Period, During-Lockdown Period and Post-Lockdown Period. This is due to the intensified traffic and industrial plumes which were operated during restricted hours. The monitoring station located at Coimbatore city - SIDCO, is an industrial area surrounded by small- scale industries. A similar scenario is being observed in Chennai city where the monitoring station is located at Manali which is an industrial area. Since the industries were allowed to operate with 50% of the workforce with restricted timings. This has a great influence on the increase in NO2 concentration during the lockdown period, a similar scenario has been observed in Chennai city [35]. It is observed, the source of NO2 is closely associated with combustion from vehicles and industries [44]. Thus, due to the prolonged exposure to NO2 causes respiratory and cardiovascular problems [45]. The above observation from the analysis highlights the need for source identification of NO2 in the zone of monitoring location and effective management of the same (Figure 5). Figure 5. Percentage Contribution of Criteria Pollutant During Different Phases of Lockdown Period. Table 4, represents the correlation matrix between rainfall and other climatic variables (Maximum Temperature, Minimum Temperature, Maximum Wind Speed, Average wind speed, and Relative Humidity). Table 4. Correlation between Rainfall and other Climatic Variables. From Table 4, it may be observed that there is a drastic change in the correlation relationship in the year 2020 compared to the year 2019. On the other hand, From Table 5 it may be observed that there is a 0.5 degree to 1-degree temperature fall during this lockdown period (phenomenon may be observed from March 2020 to May 2021). Table 5. Rainfall Characteristics for the Year 2019 and Year 2020. This fall in temperature is evident with the rise in associated relative humidity (Table 4). Figure 6, facilitates the process of visualizing the monthly rainfall for the years 2019 and 2020. Figure 6. Precipitation During the Year 2019 and 2020. From Figure 6, it may be observed that the rainfall pattern for the years 2019 and 2020 remains the same. However, in the year 2020, the precipitation has started from March with the first peak during April & the second peak during July. From Figure 6, it is inferred that there is a forward shift in the precipitation pattern during the year 2020. To further understand the shift of rainfall peak, skewness for the year 2019 is 1.35 and for the year 2020 is 0.83. The above skewness gives an indication of the early onset of monsoon in the year 2020 compared to the year 2019. From Table 6, it may be observed that in the year 2019, 36 rainy days have contributed to an annual precipitation of 601.8 mm. On the other hand, in the year 2020, annual precipitation of 719.4 mm has resulted in 43 rainy days. From the above, it may be inferred that number of rainy days is increased to contribute 19.5% higher rainfall in the year 2020 compared to 2019. Table 6. Correlation Analysis between Climatic Variables and Criteria Pollutants. On the other hand, the intensity of downpour (annual rainfall to number rainy days) remains almost the same of 16.71mm/day (the year 2019), and 16.73 mm/day (the year 2020). From the above analysis, it may be inferred that irrespective of the year 2019 (Pre lockdown) or year 2020 (during lockdown) the intensity of downpour remains constant with early onset of monsoon during lockdown with a temporal spread (across March to December of the Year 2020) of rainfall [46]. From the correlation analysis between climatic variables and criteria pollutants (Table 6), it may be observed that Temperature has a positive correlation with the criteria pollutants. Whereas, other climatic variables (relative humidity, Wind speed, and Rainfall) have a negative correlation with the criteria pollutants. From Table 6, it may be inferred SO2 & Ozone have a strong influence on all the climatic variables. From the above sentence, it may be stated that reduction in SO2 & Ozone level during the lockdown phase, resulted in decreasing trend of atmospheric temperature and thereby reflecting an increasing trend of relative humidity and rainfall [47]. Wind speed has a strong negative correlation with criteria pollutants except for CO, it states that when wind speed increases result in reduced criteria pollutant levels. During the lockdown phase, the generation of criteria pollutants is reduced thereby density of near atmosphere air reduces. Similarly, it is statistically proven that cities with high wind speed have a lesser number of COVID-19 cases [48] and it is evident that pollutant parameters have an influence on climatic variables [49]. The geographical location of Coimbatore (foothills of western ghats) creates turbulence in the wind flow through wind gusts [50], which resulted in dispersion or dilution of criteria pollutants resulting in a positive response in the microclimatic condition. Table 7 shows the Inhaled exposure dose (mg/kg/ day) for different age groups (male and female) during different phases of lockdown period. Table 7. Relationship between Male/Female Inhalation Exposure Dose. *-ve sign indicates the reduction Table 7 depicts the variation in male and female inhalation exposure dose during the different phases of lockdown scenarios. From Table 7 it may be noted that the inhaled dose trend differs with respect to age group and inflow rates. The inhaled dose for the 12-14 age group experienced maximum (both male and female) dose when compared to other age groups viz, 16-20, 21-40 and 41-19 years. A study in Coimbatore which shows that the number of persons exposed to dust is higher viz 383, where the children below the age of 10 (80%) are more likely to be affected by asthma than the adult age group [51]. This clearly shows that the air intake rate for children which is higher than the adults have great influence in the inhalation dose. Thus, the children are exposed to respiratory disease like asthma. However, pre-lockdown and post-lockdown has slight increase in inhaled exposure dose in comparison during lockdown (phase I, II, III & IV) irrespective of all the age groups. Even though the concentration is reduced in phases of lockdown, it is observed that the pattern of inhaled dose remains same viz constant decrease in percentage during different phases of lockdown. This can be clearly seen, in comparison with 12-14 (female) age group there is a decrease in 11%,21% and 24% decrease in inhaled dose for 16-20,21-40 and 41-49 (female) age group. Similarly for male there is decrease in 14%,18% and 24% for 16-20, 21-40 and 41-49 (male) age group when compared with 12-14 (male) age group. Since the air intake rate is higher for male (category 12-14 years) increases the risk to respiratory diseases like asthma, Chronic Obstructive Pulmonary Disease and even Pulmonary diseases [52]. The dosage of male (category 12-14 years) is 3% higher during pre-lockdown, and 2% higher during (P-I, II, III, IV and post lockdown) when compared to female (category 12-14 years). In conclusion, this research explores, i) whether policy decisions (lockdown) have an influence on the criteria pollutant levels, ii) influence of criteria pollutants on microclimatic condition considering the geographical location, and iii) Impact of lockdown decision on inhalation exposure dose with respect to various phases, population age group, and gender. From the results, the following are the key observations: i) Phase I & Phase II lockdown decision has a strong influence on the criteria pollutant levels. ii) Strict lockdown decision during June 2020 is reflected in the lower criteria pollutant levels during July 2020. iii) Relaxation in the operation of urban & industrial activity post-July 2020, has a direct relationship with the gradually increasing trend of criteria pollutants. iv) Reduced criteria pollutant levels have influenced the early onset of monsoon with temporal distribution of precipitation and increased number of rainy days. v) The geographical location of Coimbatore along the foothills of western ghats may have influenced the dispersion of reduced levels of criteria pollutants through wind gusts during the lockdown.vi) From the Inhalation exposure dose assessment, it is inferred that lock down decision has positive impact on reducing mg/m3 pollutant exposure. From the results, it may be concluded that the geographical location can disperse/dilute the criteria pollutants to an acceptable load/emission level. Now the research has to be more focused to define the optimal load of emission from the known point sources taking the benefit of geographical location. This may result in urban areas remaining as liveable spaces with a concept of sustainable development. Similarly, the vulnerable population is the age group of <12 years due to their high air intake volume compared to other population group. Thus, resulting into probable increase in the chance of respiratory diseases.
Data Availability
Dataset derived from public resources. These datasets were derived from the following public domain resource: https://cpcb.nic.in/ The authors thank the Central Pollution Control Board (CPCB), New Delhi, Tamil Nadu Pollution Control Board (TNPCB) for providing the air quality data and Indian Meteorological Department for providing Meteorological data. The authors declare that they have no competing financial interests that could have appeared to influence the work reported in this paper. This research did not receive any specific grant from funding agencies in the public, commercial, or not-for- profit sectors. Sneha M: Writing - Original Manuscript, Conceptualization, Methodology, Investigation, Data curation. Ramsundram N: Writing – Review & Editing, Conceptualization, Data curation, Formal analysis, Validation.
Pre-Lockdown
CC
PM10
PM2.5
NO2
SO2
CO
Ozone
PM10
1.00
0.77
-0.30
0.38
-0.03
0.57
PM2.5
0.77
1.00
0.37
0.37
-0.03
0.47
NO2
-0.32
-0.30
1.00
-0.39
0.08
-0.39
SO2
0.37
0.38
-0.39
1.00
0.24
0.63
CO
-0.03
-0.03
0.08
0.24
1.00
0.06
Ozone
0.47
0.57
-0.39
0.63
0.06
1.00
During-Lockdown
CC
PM10
PM2.5
NO2
SO2
CO
Ozone
PM10
1.00
0.88
-0.29
-0.32
-0.12
-0.07
PM2.5
0.88
1.00
-0.32
-0.42
-0.22
-0.16
NO2
-0.32
-0.32
1.00
0.73
0.47
0.34
SO2
-0.42
-0.42
0.73
1.00
0.47
0.53
CO
-0.22
-0.22
0.47
0.47
1.00
0.76
Ozone
-0.16
-0.16
0.34
0.53
0.76
1.00
Post-Lockdown
CC
PM10
PM2.5
NO2
SO2
CO
Ozone
PM10
1.00
0.89
-0.19
-0.18
0.02
0.17
PM2.5
0.89
1.00
-0.24
-0.20
-0.06
0.07
NO2
-0.24
-0.19
1.00
0.36
0.42
0.35
SO2
-0.20
-0.18
0.36
1.00
0.28
0.21
CO
-0.06
0.02
0.42
0.28
1.00
0.67
Ozone
0.07
0.17
0.35
0.21
0.67
1.00
Climatic variables
Year \ rainfall
Max Temp
Min Temp
Max wind
Average wind
Relative Humidity
2019
-0.52563
-0.11685
-0.09152
-0.02753
0.64496
2020
-0.24416
0.121914
-0.02076
-0.03958
0.484355
Year
JAN
FEB
MAR
APR
MAY
JUN
JUL
AUG
SEP
OCT
NOV
DEC
Annual
24 HRS Heaviest rainfall
Skewness
2019 monthly rainfall (mm):
0
0
0
0
19
7
1
194
42
180
125
34
601.8
120
1.4
Number of observations in the month
31
28
31
30
31
30
31
31
30
31
30
31
365
9
Number of rainy days having Rainfall more than 2.4 mm
0
0
0
0
1
1
0
6
6
12
6
4
36
8
2020 monthly rainfall (mm):
0
0.04
4
97
36
2.7
134
55
82
41
174
53.3
719.4
82
0.8
Number of observations in the month
31
29
31
30
31
29
31
31
30
31
30
31
365
10
Number of rainy days having Rainfall more than 2.4 mm
0
0
2
4
2
0
6
6
8
3
8
4
43
7
Parameters
PM2.5 (ug/m )
PM10 (ug/m )
NO2 (ug/m )
SO2 (ug/m )
CO (mg/m3)
Ozone (ug/m3)
Rainfall (mm)
-0.48
-0.46
-0.3
-0.71
-0.21
-0.63
Temperature (֯C)
0.72
0.62
0.81
0.65
0.13
0.88
Relative Humidity (%)
-0.53
-0.4
-0.81
-0.55
-0.48
-0.76
Wind speed
(kmph)
-0.88
-0.82
-0.53
-0.67
0.05
-0.88
Climatic variables and criteria pollutants
Inhalation Exposure Dose
Pre-LD
P-I
P-II
P-III
P-IV
Post-LD
Female
0
-4.84
-3.7
-3.69
-3.87
-0.97
Male
0
-3.71
-2.83
-2.82
-2.96
-0.74
Discussion and Conclusion
Acknowledgments
Disclosure Statement
Funding
Authorship contributions statement
References
Author Details
How to Cite
- Abstract viewed - 0 times
- PDF (FULL TEXT) downloaded - 0 times
- XML downloaded - 0 times