Mobile Phone Use and Brain Tumor: An Age-Period-Cohort Analysis of Brain Tumor Rates in the Nordic Population
Download
Abstract
Background: The association of the mobile phone use and risk of brain tumor remains controversial among radiation epidemiologists.
Methods: We hypothesized if an association between brain tumor and mobile phone use exists, this association will be manifested as a cohort effect (as a proxy of association between mobile phone use and brain tumor) in the incidence rates of brain tumor during the period of 1990 to 2009. We used age-period-cohort methodology (generalized log-linear model) and compared the distribution of cohort effects in the observed rates of brain tumor from 1990 to 2009 to the cohort effects from rates driven based on epidemiological study results that reported a positive association between brain tumor and mobile phone use in the Nordic population. Three latency period of 1-4 years with odds ratio (OR) of 1.2, latency period of 5-9 years with OR of 1.3, and latency period of more than 10 years with OR of 2.7 were used to estimate expected rates.
Results: The distribution of cohort effects between observed and expected rates were more similar among the males compared with females. A shorter latency was more con¬sistent to observed rates.
Conclusion: Our study supports a possible a weak association between mobile phone use and brain tumor; further fueling the controversies in association.
Introduction
The association of the mobile phone use and risk of brain tumor remains controversial among radiation epidemiologists. The International Agency on Research in Cancer has categorized the exposure as a possible human carcinogen (group 2b) [1]. The association of brain tumor and mobile phone use has been studied in experimental (animal), epidemiologic (human), and ecological (incidence trends) studies. These studies have reported controversial results. Animal studies have reported increased markers of carcinogenicity such as DNA damage [2, 3, 4] and oxidative stress [5] but no risk of cancer [6] among laboratory animals when they have been exposed to radiation dose equal to mobile phone. Epidemiologic studies are more controversial and inconclusive compared to animal studies. While some epidemiologic studies reported high relative risks as high as 3.9 in magnitude [7] some studies have reported even protective effects for certain type of brain tumors [8, 9, 10, 11, 12]. The controversy on epidemiologic studies has been mainly blamed on the design and especially on the recall bias as many of the epidemiologic studies were case control studies and exposure were ascertained by interviewing subjects [13, 14, 15, 16]. The ascertainment of exposure has also been complicated with fast changing of technology such as introduction of hands free devises [16]. With lots of methodological uncertainties in epidemiologic studies, there are some positive indications supporting a causal effect such as; consistency in reporting of higher risk for glioma, a higher risk for proximity of tumor to the source of radiation such as tumor of temporal lobe and the cerebellum,9; [17] and a dose response consistency of higher risk in upper deciles of exposure [18]. The third line of Studies has been more of ecological nature by looking at the trend of brain or subcategories of brain cancers in the population that maintained quality cancer registry like Nordic countries, USA and UK. These studies have mainly focused on the trend and the changing of incidence rates over time; expecting an increase in incidence after 1990 when the widespread use of mobile phone was started. None of the incidence and trend studies concluded a positive association between mobile use and brain cancer.
The aim of this study is to utilize the more flexible age-period-cohort (APC) analysis to look for possible effect of a positive association between mobile phone use and brain tumor as a legitimate cohort effects on brain tumor rates during 1990 to 2009 (time that mobile phone use was widespread). We hypothesized that if a positive association exists, the cohort effects in the observed incidence rates of brain cancer after 1990 would correspond in terms of distribution and magnitude to the cohort effects on the expected rates based on the published epidemiological studies’ results.
Materials and Methods
In this study: first, we estimated expected incidence rates using the prevalence of mobile phone use and relative risk of mobile phone and brain tumor from published literatures; second we applied age period cohort model to the expected incidence rates and the observed incidence rates reported by the registries; and third, we compared the cohort effects measured in observed rates to cohort effect measured in expected rates. Followings detail the materials and methods used in the study.
Retrieved results from the two databanks were compared and duplicates were discarded. Because the searches were not restricted (for example, for field, year, language, country, or study location, etc), a large number of articles were found, and their abstracts (that had been prepared by End Note and Reference Manager software programs) were entered into the data bank of the article. Then, with the aim to separate and eliminate irrelevant results, further limited search was conducted in this databank. Irrelevant abstracts were removed from databank. Next, through review of abstracts of the remaining articles, initially 100 articles, with more relevance were selected. Then, through more detailed review, eventually 19 articles with possibility of extracting main components of an educational relationship were used for final analysis.
The observed rates: The yearly number of brain tumors (ICD 10 coded C71.X, and C72.X) and Their corresponding population numbers based on five-year age group and sex for Nordic Countries (Sweden, Finland, Denmark, Norway, and Island) for the period of 1990 to 2009 were obtained from the Association of Nordic Cancer Registries by a request that included the title and the aim of the study. Matrix of rates (hereafter called ob served rates) for each sex was constructed for 13 five-year age groups (15 to 75 years) and 19 yearly periods (1990 to 2009).
The epidemiologic studies: We identified all the original epidemiologic studies (case control or cohort) assessing the association of mobile phone use and brain tumor from the Medline. We used mobile, cellular, brain tumor, neoplasm, meningioma, and glioma as search terms. We identified 15 studies (Appendix I) (Fig. 3). Since we had data just for brain as whole, we used studies that just reported odd ratio (OR) or rate ratio (RR) for brain tumor. We did not used studies that reported odd ratio or rate ratio for glioma or other subcategories of brain tumor.
Figure 3 :(Appendix I)
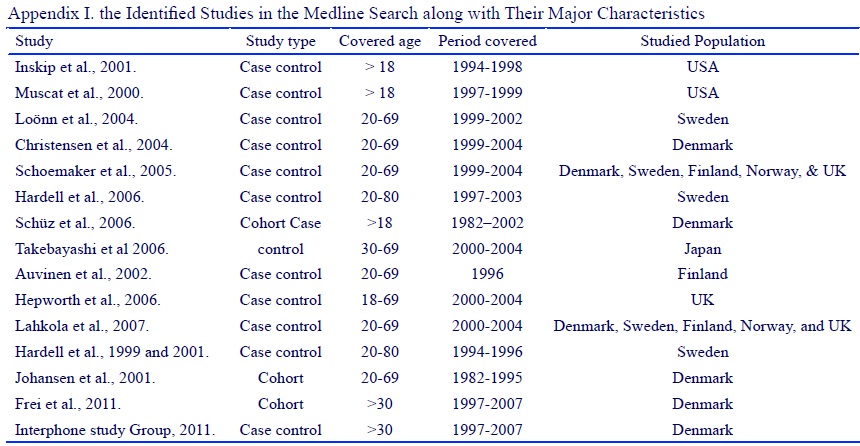
Among the studies that reported odd ratio for brain tumor (Tabl 1), three studies reported a positive association with almost similar magnitude and the resting reported either a protective or no association. We used the Hardell, et al [19] study that reported odds ratio of 1.1 for a latency period of 1-4 years, 1.2 for a latency period of 5-9 years, and 2.5 for a latency period of more than 10 years to estimate the expected rates based on epidemiologic study results.
Study | Population | Tumor | RR/OR (95% CI) | Reference No. |
Muscat et al. | USA | Brain cancer | 0.74 (0.50 to 1.10)* | 9 |
Inskip et al. | USA | Brain cancer | 0.8 (0.6 to 1.1)* | 10 |
Auvinen et al. | Finland | Brain cancer | 1.3 (0.4 to 4.7)* | 8 |
Schüz et al. | Denmark | Brain cancer: male | 0.96 (0.87 to 1.05) | 11 |
Hardell et al. | Sweden | Brain cancer: female Brain cancer | 1.03 (0.82 to 1.26) 2.7 (1.6 to 4.7) | 7 |
Hardell et al. | Sweden | All malignant brain: 1-4 year latency All malignant brain: 5-9 year latency | 1.1 (0.9 to 1.4)* 1.2 (0.9 to 1.5)* | 19 |
a) All malignant brain: ≥10 year latency 2.5 (1.8 to 3.3), b) Adjusted for sex and age
The prevalence of exposure: The prevalence of mobile phone use was determined using data for mobile subscriptions for Sweden, Finland, Denmark, and Norway, from the ITU (International Telecommunication Union) web site for duration of the study period, 1990 to 2009 (Fig. 1).
Figure 1 :The Distribution of Mobile Subscriptions. In The Study Population During The Study Period.
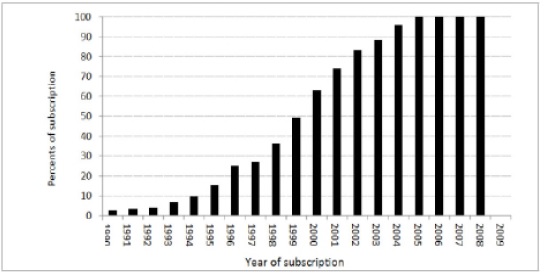
a) Data were extracted from International Telecommunication Union online resources at :
http://www.itu.int/ITU-D/ict/statistics/explorer/index.html
The ITU reported subscription for the whole population without reference to age and sex. The age and sex distribution of mobile phone use reported for a cohort of Swedish population for the year of 1990 and 1995 were used to estimate the prevalence of exposure based on sex, and age. The prevalence of exposure was estimated based on the three scenarios of latency period as reported among the controls from the same study that provided the odds ratios. Tabl 2 presents the distribution of exposure prevalence (averaged over five-year age interval) for different latency scenarios and sex used in the study.
Year | Male | Female | ||||
Latency scenarios | Latency scenarios | |||||
1-4 year | 5-9 year | >10 year | 1-4 year | 5-9 year | >10 year | |
1990 | 0.13 | 0.06 | 0.03 | 0.13 | 0.06 | 0.03 |
1995 | 0.71 | 0.35 | 0.14 | 0.44 | 0.22 | 0.09 |
2000 | 2.86 | 1.41 | 058 | 2.24 | 1.10 | 0.46 |
2005 | 4.54 | 2.23 | 0.92 | 4.34 | 2.13 | 0.88 |
2009 | 4.54 | 2.23 | 0.92 | 4.54 | 2.23 | 0.92 |
The expected rates based on epidemiologic studies: To estimate the rates based on epidemiologic studies; the Levin’s formula was used to estimate the excess number of cases for different scenarios of latency period. For this purpose, we estimated the baseline age specific rates (age specific rates in the absence of observed cohorts and period effects) from the APC model. Then, we estimated the excess rates attributed to the exposure scenarios for different age groups and years. Finally we added the excess rates to the baseline age specific rates to obtain the Expected rates (hereafter expected rates).
Statistical Analysis
The age-period-cohort model: for this model, the periods were constructed in intervals of 1 years (19 intervals for the entire period) and the five-year age groups were truncated to age more than 15 and less than 75 (13 age groups). The constrained generalized linear model (CGLM), the most utilized approach in epidemiology literature dealing with age-period cohort analysis was used. For this purpose, a log-linear model with the general form that includes a (age), p (period), c (cohort) as follow were applied:
Log[λ(a,p)] = f(a) + g(p) + h(c),
Where a, p and c represent the mean age, period and cohort and f, g, h are parametric functions fitted to the data. In this model, in addition to the main effect of age, the model allows estimation of other components contributing to magnitude of rates specially the secular changes of rate across studied period and birth cohorts. The secular change corresponds, interchangeably, to hazard due to period or cohort and it has been utilized by others as annual percent change of rates over period of time, or net drift. The net drift represents the estimated annual percent change (EAPC) in the rates over the passage of time. The model was used 1) to estimate the net drift or EAPC, 2) to estimate the age specific rates (adjusted for period and cohort effects) for each year of the study period, and 3) to estimate the cohort effects for the observed rates as well as the expected rates (estimated rates based on prevalence of exposure and magnitude of risk). The priority assumption for parameterization was that mainly cohort effect explains the changing of rates across aging intervals for each period (one year period) in the study population. In this parameterization, the model estimates the age effect as log of rate, the cohort effect as log of rate ratio relative to a reference cohort (here cohort born in 1915) while period effect constrained to be zero on average with zero slopes. For details of how the parameterization works please refer to “age-period-cohort models for the Lexis diagram” by B. Carstensen [20]. Data were analyzed using the R 2.14.1 statistical software utilizing Epi 1.1.9 package (R Development Core Team, 2009).
The observed five years average rates were compared to the expected five years average rates using rate ratios and their confidence intervals as measure of statistical significance. Statistical judgment of comparing the expected cohort effects to observed cohort effects was based on the 95% confidence interval around estimated cohort effects for each cohort and scenario of exposure.
Results
A total of 58890 cases (26882 male and 32008 female) of brain tumors over the period of 1990 to 2009 (inclusive) were included in the analysis. The trend in incidence measured as net drift was 2% for females and 1% for males during the study period. Comparing the observed with the expected rates, there were no statistically (expressed as 95% confidence interval around the rate ratios of expected to observed rates) significant differences between the observed and the expected for both males and females. Tabl 3 presents the rates averaged over five years; the rate ratios and the confidence intervals of the rate ratios based on sex for different scenarios of exposure.
Y | Observed Rates | Latency period 1-4 years | Latency period 5-10 years | Latency period > 10 years | ||||
Expected rates | Rate Ratio (95% CI) | Expected rates | Rate Ratio (95% CI) | Expected rates | Rate Ratio (95% CI) | |||
1990-1995 | 12.95 | 13.00 | 1.005 (0.97, 1.05) | 13.00 | 1.000 (0.97, 1.03) | 13.01 | 1.001 (0.97, 1.03) | |
1996-2000 | 13.65 | 13.68 | 1.001 (0.97, 1.04) | 13.68 | 1.000 (0.97, 1.04) | 13.72 | 1.003 (0.97, 1.04) | |
2001-2005 | 14.17 | 14.26 | 1.007 (0.97, 1.04) | 14.26 | 1.007 (0.97, 1.04) | 14.55 | 1.028 (0.99, 1.06) | |
2006-2009 | 14.91 | 15.00 | 1.006 (0.97, 1.04) | 14.99 | 1.006 (0.97, 1.04) | 15.10 | 1.014 (0.98, 1.05) |
The cohort effect showed similar pattern as the incidence showed. The overall cohort effects from estimates based on epidemiologic finding was similar to cohort effect estimated from observed data. This similarity was more evident among older cohorts compared to younger cohorts and more among males compared to females. Among the males, there was higher cohort effects for those born after 1980 in expected rates compared to cohort effects in the observed rates. For the 1-4 year latency period, the cohort effect in the expected rates was 1.34 with 95% CI of 1.15 to 1.55 for those born in 1980, 1.40 with 95% CI of 1.15 to 1.70 for those born in 1990, and 1.31 with 95% CI of 1.13 to 1.80 for those born in 1994. These figures were 1.33 with 95% CI of 1.14 to 1.55 for born in 1980, 1.32 with 95% CI of 1.09 to 1.61 for the year 1990, and 1.31 with 95% CI of 1.04 to 1.65 for the year 1994 for observed rates. For the 5- 10 years latency period, the cohort effect in expected rates was 1.34 with 95% CI of 1.15 to 1.55 for those born in 1980, 1.40 with 95% CI of 1.15 to 1.70 for those born in 1990, and 1.43 with 95% CI of 1.13 to 1.80 for those born in 1994. These figures in the observed rates were 1.33 with 95% CI of 1.14 to 1.55 for born in 1980, 1.32 with 95% CI of 1.09 to 1.61 for the year 1990, 1.31 with 95% CI of 1.04 to 1.65 for the year 1994. For > 10 years latency period, the cohort effect in the expected rates was 1.38 with 95% CI of 1.19 to 1.61 for those born in 1980, 1.46 with 95% CI of 1.20 to 1.77 for those born in 1990, and 1.49 with 95% CI of 1.18 to 1.87 for those born in 1994. These figures in the observed rates were 1.33 with 95% CI of 1.14 to 1.55 for those born in 1980, 1.32 with 95% CI of 1.09 to 1.61 for those born in the year 1990, 1.31 with 95% CI of 1.04 to 1.65 for those born in the year 1994. Among the Female population, the cohort effects between the observed and expected rates were slightly different especially for those born after 1980 but the difference was not statistically significant. For the 1-4 yeas latency period, the observed cohort effect was 2.76 with 95% CI of 2.38 to 3.19 for those born in 1980, 3.22 with 95% CI of 2.65 to 3.92 for those born in 1990, and 3.43 with 95% CI of 2.72, 4.33 for those born in 1994. These figures were 2.67 with 95% CI of 2.31 to 3.10 for born in 1980, 2.82 with 95% CI of 2.31 to 3.44 for the year 1990, 2.84 with 95% CI of 2.24 to 3.61 for the year 1994 for the observed rates. For the 5 years latency period, the cohort effect among the expected rates was 2.76 with 95% CI of 2.38 to 3.19 for those born in 1980, 3.22 with 95% CI of 2.65 to 3.92 for those born in 1990, and 3.43 with 95% CI of 2.2.72 to 4.33 for those born in 1994. These figures were 2.67 with 95% CI of 2.31 to 3.10 for born in 1980, 2.82 with 95% CI of 2.31 to 3.44 for the year 1990, 2.84 with 95% CI of 2.24 to 3.61 for the year 1994 for the observed rates. For ten year latency period, the cohort effect for expected rates was 2.85 with 95% CI of 2.46 to 3.29 for those born in 1980, 3.36 with 95% CI of 2.76 to 4.08 for those born in 1990, and 3.58 with 95% CI of 2.84 to 4.52 for those born in 1994. These figures were 2.67 with 95% CI of 2.31 to 3.10 for born in 1980, 2.82 with 95% CI of 2.31 to 3.44 for the year 1990, with 95% CI of 2.24 to 3.61 for the year 1994 for observed rates. Fig .2 presents the distribution of cohort effects for different scenarios of latency periods for both males and females.
Figure 2 :The cohort effects for both males and females. Graph A for latency 1 to 4 years, B for latency 5 to 10 years, C for latency more than 10 years. The solid line presents the cohort effects for observed and the dotted line presents the cohort effects for the expected rates.
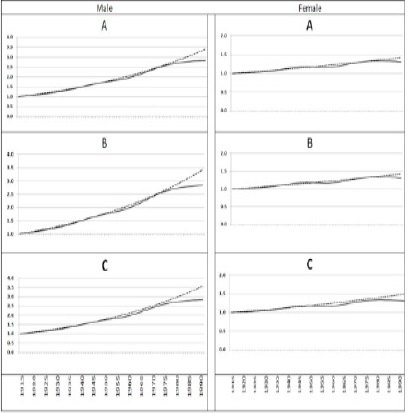
Appendix II (Fig.4) and III (Fig.5) present the numerical values of the cohort effects and their confidence intervals for different scenarios of latency period for females and males, respectively.
Figure 4 :Appendix II
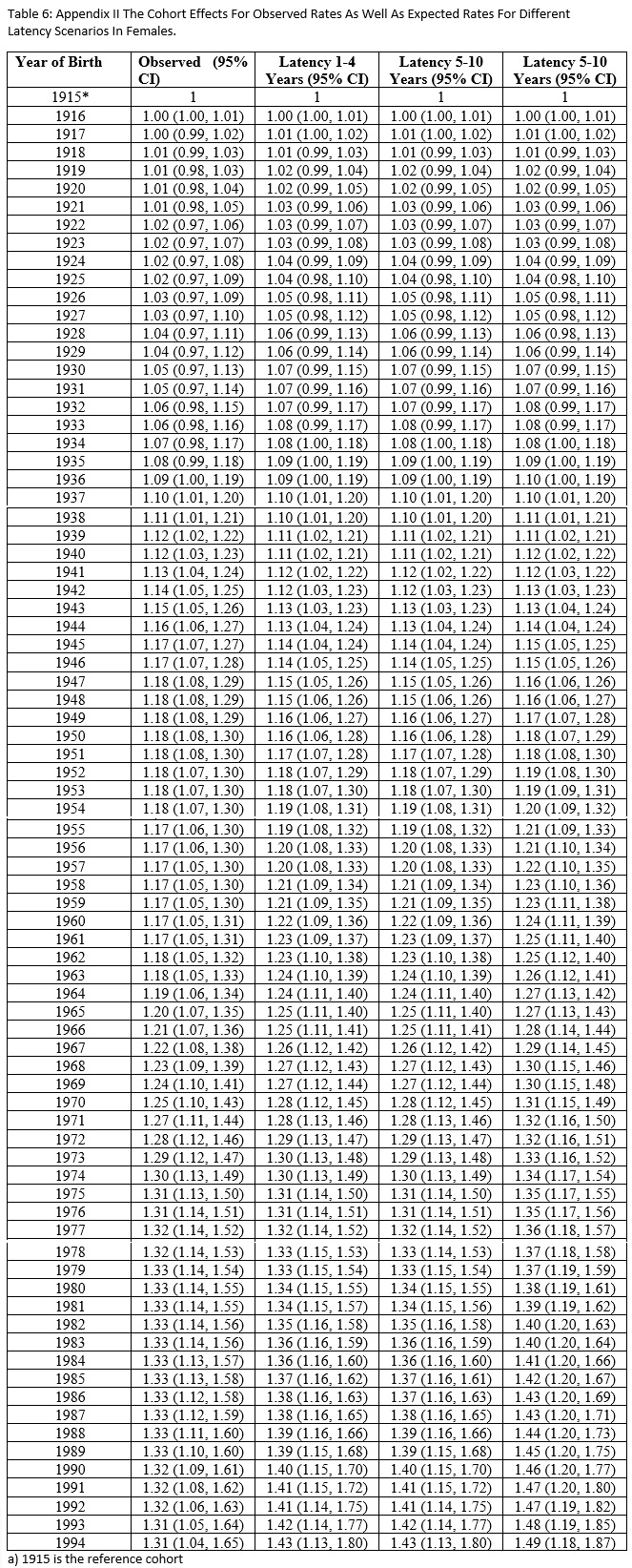
Figure 5 :Appendix III
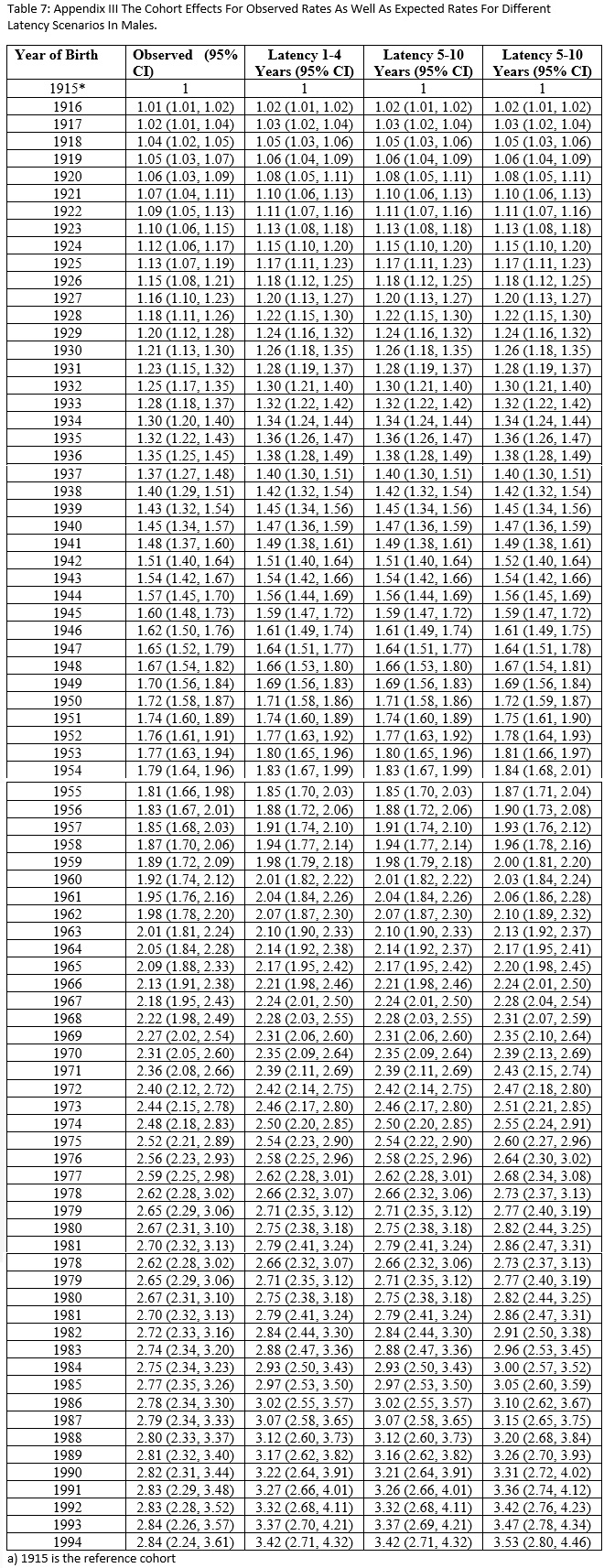
Discussion
Our study proved that the expected rates (the expected rates were estimated based on a positive association between mobile phone use and brain tumor) and their corresponding cohort effects were similar to the observed rates and observed cohort effects. The similarity was more evident among males than females. Studies that have addressed association of mobile phone use and brain tumor using incidence rates are divided into two groups 1) those that used just trends in observed rates and compared the rates before the widespread use of mobile phone (1990) with the rates after widespread mobile phone use (after 1995), and 2) those that utilized epidemiologic study results and estimated rates based on reported relative risks and prevalence of mobile use as our study did. In the first group, a study on Nordic [21] countries used joint point regression analyses of incidence rates of both glioma and eningioma from 1974 to 2003 and concluded no association between mobile phone use and incidence of central nervous system tumors and then the same group updated their study [22] covering up to the year 2008 and concluded the same. Another study of incidence trend in USA population [23] did not report increase of incidence from 1992 to 2006 except in the females of 20-29 years age group but the study concluded no association between mobile use and brain tumor. Similar study [24] in the United Kingdom concluded that the increased use of mobile phones between 1985 and 2003 has not led to an increase in the incidence of brain tumor in England. Among the group of studies that used the results of epidemiological studies to simulate rates and develop an expected rates attributed to mobile phone use, a study in the Nordic population [22] used relative risk 2.0 for all users for an latency period up to 15 years, RR of 1.5 for latency up to 10 years, and RR of 1.2 for latency up to 5 years and concluded no definite association but reported a higher likelihood of association in higher magnitude of rate ratios and shorter latency period as a possibility. Another [25]study in US population, which used relative risks of glioma with different latency periods and cumulative hours of phone use as exposure, predicted rates that were 40% higher than the observed rates. In all of the studies that were mentioned, there was a consistency that all reported a slight increase in observed incidence (not a sharp increase) and concluded that the increase in incidence did not correspond to the increase in the mobile phone use. While the conclusion based on inconsistency in correlation between mobile phone use and incidence rates stands, but if the association between mobile phone use and the brain tumor is a weak association and/or the association exists when the exposure is very dense (higher deciles of exposure resulting in lower prevalence of exposure) then the expectation of high correlation between change in incidence and mobile phone cannot be easily realized. In another word, for situation that the association is very weak or the association is strong but the prevalence of exposure is very low (this can happen when association is related to higher doses of exposure) detection of the association between exposure and outcome cannot be easily detected from trend or before and after incidence comparisons. Our study showed that the pattern and magnitude of cohort effect (an effect that is attributed to differential effect of exposure to different age groups) was similar between observed rates and expected rates. As a cohort effect is a function of how an exposure affects different age groups and in the light of the fact that a positive association between mobile phone use and brain tumor has been suggested in higher intensity of exposure, result of our study tends to support the association of mobile phone use and brain tumor. The fact that we used cohort effect as a proxy of association between mobile phone use and brain tumor has its own merit among the epidemiologists. There is a widespread consensus among epidemiologists to attribute the changes of rate in cancer over a period time to a cohort effect rather than period effect when the nature of association is etiologic
The methodology we used is very established and routine way of analyzing rates at population level when the data of aggregate nature and calendar time is involved. We used the model not only to estimate cohort effects but also to estimate based line incidence for each year and age group. The age-period-cohort analysis has been a major tool in the hands of demographers and in recent decades frequently utilized by epidemiologists. The methodology, while very common in use suffers major problem especially when it is utilized to attribute the underlying cause of changes of rates to period versus cohort (the non-identifiability problem). The non- identifiably problem was not relevant in our study as we assigned the change of rates to cohort effect as it was our assumption.
There are several limitations in our study that need consideration. Using brain tumor instead of glioma as outcome when epidemiologic studies normally support association between mobile use and glioma, can be considered as a limitation for our study. This limitation can be partly recovered for two reasons: 1) it has been reported that meningioma rates have been stable during the study period in our study population hence the changing of rates in our study tends to be attributed to glioma, and 2) the fact that more than 70 percents of brain tumors are gliomas [21, 22] tends to support our conclusion. Another limitation of our study is the fact that we used the age distribution of mobile phone use for the year 1995 and apply it to other years.
This extrapolation of age distribution may, in fact, affect our result especially in older age groups (more than 50 years). The distribution of mobile phone use in the study population had increased from 30% in 1995 to 100% in 2005 (Fig. 1). Such increase would include older people and would change the age specific distribution of mobile phone toward more homogeneity in age specific usage of mobile phone. However, the intensity of mobile phone use may not change and may still differ by age (higher intensity of exposure among younger compared to older population). Another major limitation of our study is the fact that the expected rates were driven based on relative risks from epidemiologic studies when there are lots of inconsistencies in magnitude of association between mobile phone use and brain tumor in epidemiologic studies.in Conclusion,Our study support possible association between mobile phone and brain tumor.We demonstrated that the expected cohort effects driven from epidemiological study findings correspond to the observed cohort effects in an exposed population; high lighting the need for more surveillance of mobile phone exposure and brain tumor.
Conflict of interest:
No conflict of interest
Acknowledgements
The authors thank the Association of Nordic Cancer Registries for providing the data for this analysis.
References
[1]. Baan R, Grosse Y, Lauby-Secretan B, El Ghissassi F, Bouvard V, Benbrahim-Tallaa L, et al. Carcinogenicity of radiofrequency electromagnetic fields. Lancet Oncol. 2011;12(7):624-6.
[2]. Habash RW, Elwood JM, Krewski D, Lotz WG, McNamee JP, Prato FS. Recent advances in research on radiofrequency fields and health: 2004-2007. J Toxicol Environ Health B Crit Rev. 2009;12(4):250-88.
[3]. Trosic I PI, Milkovic-Kraus S, Mladinic M, Zeljezic D. Effect of electromagnetic radiofrequency radiation on the rats’ brain, liver and kidney cells measured by comet assay.Coll Antropol. 2011;35(4):1259-64.
[4]. Yang L, Hao D, Wang M, Zeng Y, Wu S, Zeng Y. Cellular neoplastic transformation induced by 916 MHz microwave radiation. Cell Mol Neurobiol. 2012;32(6):1039-46.
[5].Guler G, Tomruk A, Ozgur E, Sahin D, Sepici A, Altan N, et al. The effect of radiofrequency radiation on DNA and lipid damage in female and male infant rabbits. Int J Radiat Biol. 2012;88(4):367-73.
[6]. La Regina M, Moros EG, Pickard WF, Straube WL, Baty J, Roti Roti JL. The effect of chronic exposure to 835.62 MHz FDMA or 847.74 MHz CDMA radiofrequency radiation on the incidence of spontaneous tumors in rats. Radiat Res. 2003;160(2):143-51.
[7]. Hardell L, Carlberg M, Mild KH. Case-control study of the association between the use of cellular and cordless telephones and malignant brain tumors diagnosed during 2000-2003. Environmental research. 2006;100(2):232-41.
[8]. Auvinen A, Hietanen M, Luukkonen R, Koskela RS. Brain tumors and salivary gland cancers among cellular telephone users. Epidemiology (Cambridge, Mass). 2002;13(3):356-9.
[9]. Muscat JE, Malkin MG, Thompson S, Shore RE, Stellman SD, McRee D, et al. Handheld cellular telephone use and risk of brain cancer. Jama. 2000;284(23):3001-7.
[10]. Inskip PD, Tarone RE, Hatch EE, Wilcosky TC, Shapiro WR, Selker RG, et al. Cellular-telephone use and brain tumors. The New England journal of medicine. 2001;344(2):79-86.
[11]. Schuz J, Bohler E, Berg G, Schlehofer B, Hettinger I, Schlaefer K, et al. Cellular phones, cordless phones, and the risks of glioma and meningioma (Interphone Study Group, Germany). Am J Epidemiol. 2006;163(6):512-20.
[12]. Johansen C, Boice J, Jr., McLaughlin J, Olsen J. Cellular telephones and cancer--a nationwide cohort study in Denmark. J Natl Cancer Inst. 2001;93(3):203-7.
[13]. Vrijheid M, Armstrong BK, Bedard D, Brown J, Deltour I, Iavarone I, et al. Recall bias in the assessment of exposure to mobile phones. J Expo Sci Environ Epidemiol. 2009;19(4):369-81.
[14]. Vrijheid M, Deltour I, Krewski D, Sanchez M, Cardis E. The effects of recall errors and of selection bias in epidemiologic studies of mobile phone use and cancer risk. Journal of exposure science & environmental epidemiology. 2006;16(4):371-84.
[15]. Vrijheid M, Cardis E, Armstrong BK, Auvinen A, Berg G, Blaasaas KG, et al. Validation of short term recall of mobile phone use for the Interphone study. Occupational and environmental medicine. 2006;63(4):237-43.
[16]. Swerdlow AJ, Feychting M, Green AC, Leeka Kheifets LK, Savitz DA. Mobile phones, brain tumors, and the interphone study: where are we now? Environmental health perspectives. 2011;119(11):1534-8.
[17]. Brain tumour risk in relation to mobile telephone use: results of the INTERPHONE international case-control study. International journal of epidemiology. 2010;39(3):675-94.
[18]. Hardell L, Carlberg M, Soderqvist F, Mild KH, Morgan LL. Long-term use of cellular phones and brain tumours: increased risk associated with use for > or =10 years. Occupational and environmental medicine. 2007;64(9):626-32.
[19]. Hardell L, Carlberg M, Hansson Mild K. Pooled analysis of two case-control studies on use of cellular and cordless telephones and the risk for malignant brain tumours diagnosed in 1997-2003. International archives of occupational and environmental health. 2006;79(8):630-9.
[20]. Rosenbauer J, Strassburger K. Comments on 'age-period-cohort models for the Lexis diagram' by Carstensen B. Statistics in Medicine 2007; 26:3018-3045. Stat Med. 2008;27(9):1557-61; author reply 61-4.
[21]. Deltour I, Johansen C, Auvinen A, Feychting M, Klaeboe L, Schuz J. Time trends in brain tumor incidence rates in Denmark, Finland, Norway, and Sweden, 1974-2003. J Natl Cancer Inst. 2009;101(24):1721-4.
[22]. Deltour I, Auvinen A, Feychting M, Johansen C, Klaeboe L, Sankila R, et al. Mobile phone use and incidence of glioma in the Nordic countries 1979-2008: consistency check. Epidemiology (Cambridge, Mass). 2012;23(2):301-7.
[23]. Kohler BA, Ward E, McCarthy BJ, Schymura MJ, Ries LA, Eheman C, et al. Annual report to the nation on the status of cancer, 1975-2007, featuring tumors of the brain and other nervous system. J Natl Cancer Inst. 2011;103(9):714-36.
[24]. de Vocht F, Burstyn I, Cherrie JW. Time trends (1998-2007) in brain cancer incidence rates in relation to mobile phone use in England. Bioelectromagnetics. 2011;32(5):334-9.
[25].Little MP, Rajaraman P, Curtis RE, Devesa SS, Inskip PD, Check DP, et al. Mobile phone use and glioma risk: comparison of epidemiological study results with incidence trends in the United States. BMJ (Clinical research ed). 2012;344:e1147.
[26]. Keyes KM, Utz RL, Robinson W, Li G. What is a cohort effect? Comparison of three statistical methods for modeling cohort effects in obesity prevalence in the United States, 1971-2006. Social science & medicine (1982). 2010;70(7):1100-8.
License
Copyright
© ,
Author Details