Accelerating Breast Cancer Biomarker Discovery by Mass Spectrometry Proteomics and Current Bioinformatics Tools
Download
Abstract
Across the globe, we can continue to observe a rise in the prevalence of breast cancer. The World Health Organization registered 600,000 cases of death due to breast cancer in 2021 and estimated that there would be 1 in every 8 women diagnosed with breast cancer in the next 10 years. Studies have discovered the role of proteins in the pathways that affect breast cancer but have not found a potential biomarker effective for all types, especially for triple-negative breast cancer. It remains a challenge to detect and treat breast cancer, especially if found in the later uncurable stage. With this, proteomics becomes a practical approach to screening new protein biomarkers for breast cancer diagnosis, therapy, and disease control. Proteomics covers the study of the entire protein, and its modification has been leading the race in breast cancer biomarker discovery made possible through the advancement of mass spectrometry and various bioinformatics tools. The combination has brought novel information being the fastest yet simplest approach for deep, comprehensive, and high throughput approach. This review article will give an overview of these trending online tools for proteomics research while citing examples of their utility with known clinical breast cancer biomarkers.
Introduction
Across the globe, we can continue to observe a rise in the prevalence of breast cancer. The World Health Organization registered about 600,000 cases of death due to breast cancer and estimated that 1 in every 8 women (12.9%) would be diagnosed with breast cancer in the next 10 years [1]. Studies have discovered the role of proteins in the pathways that promote or inhibit breast cancer yet have not successfully delivered a universal biomarker for all breast cancer types, especially for triple-negative breast cancer. It is still a challenge to detect and treat various types of breast cancer, primarily if it is found in the later uncurable stage. Many countries, particularly in the Philippines, have placed a spotlight to support research on breast cancer being its most prevalent cancer among women [2]. It is becoming an alarming concern because of a recent WHO commentary forecast stating that by the year 2030, the cancer rate in developing countries will rise exponentially. With this, proteomics becomes a practical approach to screening new protein biomarkers for breast cancer diagnosis, therapy, and disease control. Proteomics covers the study of the entire protein, and its modification has been leading the race in breast cancer biomarker discovery. Biomarker discovery is made possible through the advancement of mass spectrometry (MS) instrumentation and the various bioinformatics tools that this modern technology offers. The combination has brought novel information being the fastest yet simplest approach for deep, comprehensive, and high throughput proteomic biomarker research. This article will give an overview of the most sophisticated tools for proteomics research that can be applied in breast cancer research and other diseases.
The Proteomics Workflow
Figure 1 is a typical MS proteomic workflow that incorporates improved data acquisition methods and peptide-centric analysis, creating a highly confident estimate of the peptide sequence under investigation.
Figure 1. Proteomics Workflow. Combined technology of mass spectrometry and current bioinformatics tools using nanoscale sample is a high-throughput and reproducible method for accurate identification and quantification of novel protein biomarkers for breast cancer.
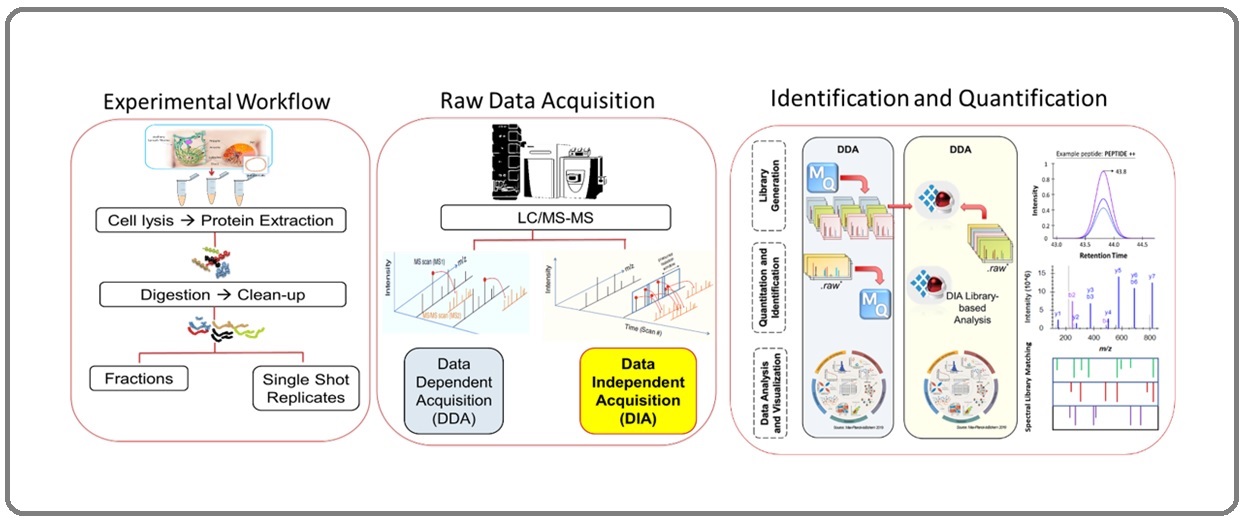
Mass spectrometry is the heart of this method, it is in tandem with liquid chromatogram (LC-MS), and together they create a very powerful tool in proteomics discovery. Mass spectrometry is continuously being upgraded to combine powerful technologies and computing systems to enhance its ability to measure accurately and comprehensively isotopic abundances, atomic and molecular masses of fragment ions to identify the mass and structure of peptides and proteins [3]. These proteins can be sourced from complex biological samples like fresh tissues, FFPE tissues, body fluids, and cell lines (Figure 1). The liquid chromatogram system injects the digested protein samples eluates into the mass spectrometer by first converting them into precursor ions by collision with an intense beam of electrons. These precursor ions passing through the MS1 analyzer enter another collision cell where they are broken further into smaller fragments to form ions carrying a +1 or +2 charge. The fragment ions will travel in a vacuum at a particular time of flight (TOF) before they reach the ion detector in the instrument. Heavier fragments will travel with a shorter time of flight than lighter fragments; therefore, lighter fragment ions will reach the ion detector earlier. The MS analysis results in a high-resolution plot of mass to charge ratio (m/z) and the relative abundance of the isotopic peaks. These spectra are used for protein structure elucidation and protein identification of the components of the sample. MS and the recent bioinformatics tools make it possible to automate peptide sequencing and create proteome profiling databases that fast-track the discovery of promising biomarkers for breast cancer.
Mass Spectrometry-Based Proteomics Software
Mass spectrometry proteomics software was developed in the early 1990s with specially curated search engines. To date, Mascot [4, 5], Andromeda [6], and Pulsar for Spectronaut [7] are the most advanced software capable of handling enormous and convoluted datasets. In every developed software, we can find sequence database search engines that perform high throughput matching of the proteomic spectra to peptides and protein groups sequencing and identification.
An MS spectrum is acquired either by data-dependent acquisition (DDA) or data-independent acquisition (DIA) (Figure 1) [8]. In DDA, peptide precursors ions are scanned, but only the highest intensities at the MS mass analyzer (MS1) will be selected for further fragmentation for the sequential MS mass analysis (MS/MS or MS2). The DDA method may exclude low intensities but significant fragments. Some fragments become ejected out in a trajectory and will never reach the detector for measurement in the next mass analyzer (MS2). Some important precursor ions are lost at the MS2 analysis, creating some gaps later in quantitation. As a result, some peptides get low scores, and their p-value higher than α > 0.05.
On the other hand, the DIA method scans the entire mass spectrum produced from MS1 ion intensities and is further fragmented in the MS2 mass analysis. All precursor ions are selected sequentially over this method’s very narrow isolation window. These ions are fragmented over pre-selected smaller ranges of precursor m/z ratio range. Then MS1 precursor ions enter the collision cells before reaching the MS2 mass analyzer. From either DDA or DIA methods, we collect the experimental raw mass spectrum of the samples from the MS analysis. The MS spectra are processed in search engines and converted into proteome structure, protein IDs, and visual plots. This is the most laborious part of the workflow because of the enormous mass spectra for processing. However, current state-of-the-art computer software has been developed to address this challenge. Bioinformatic tools and their well-crafted algorithm performs quick calculations to give scores relative to an accurate matching of mass spectra to amino acid sequences of a peptide from the database. The MS score indicates confident peptide to database matching; thus, those with high scores will be statistically significant and included in the analysis summary.
To process the raw dataset, the MS and MS/MS raw files are first converted into peak data lists before they are directly uploaded to Mascot (http://www.matrixscience. com/) for interactive searching in the combined FASTA format and public sequence databases like Swiss-Prot [9]. Accurate and efficient detection of the MS1 peaks, or MS2 multiple spectra, is critical for a successful peptide database engine search. Parameters used in the benchtop laboratory preparation of samples and protein standards and conditions for database search are encoded in the web-based interactive search form of Mascot. The parameters included are the enzyme/s used for digestion, fixed and variable modifications of the amino acid residues, and the rest are the other default settings for Mascot peptide mass fingerprinting. The biological sequence comparison algorithms in Mascot establish an automated protein identification, characterization, and quantification. Mascot utilizes one or several peptide masses and fragment ion data to create structures and sequence information, standard sequence tags, and error-tolerant sequence tag, among other valuable details provided in the program. Mascot also features its performance validation utilizing a target-decoy search at a 1% false discovery rate (FDR), significant match to at least 2 distinct sequences, and probability-based score to the order of 70 for each peptide sequence.
Maxquant (https://maxquant.net/maxquant/) is another proteomics software developed to address the challenges of analyzing extremely large raw mass spectrum data from various LC-MS technologies. The software can perform quantification with or even without the addition of stable labeling isotopes into peptide samples before its MS analysis [10]. Andromeda is the search engine for the Maxquant proteomics software developed by the Cox laboratories [11] in Germany. Maxquant begins its process with the features provided in the MS spectra, such as mass per charge ratio, intensity or abundance of the peptide, and the retention time. Maxquant pools these data and converts it into a three-dimensional MS spectrum. Peaks from the MS spectra are grouped, forming isotopic patterns to detect the slightest mass or charge state differences between two co-eluting or overlapping peptides. The analysis in Maxquant shows the MS/MS spectrum from the peak outputs of fragment ions, the derived protein sequence, mass chromatogram, and the 3D isotopic peaks. Protein groups and peptide identification and quantification in Maxquant are downloadable in tab-separated tables, full details on modification-specific data, evidence at the peptide level, and MS/MS tables. The Maxquant search results can then be uploaded directly to the Maxquant Perseus software (https://maxquant.net/ perseus/) for a multifaceted statistical interpretation of the proteome quantification, post-translational modifications and biological annotations for Homo sapiens and other species. Perseus can perform a high-dimensional omics statistical data analysis, including normalization, multiple hypothesis testing, correlation, and produces publication-ready visual outputs from any text (.TXT) or comma-separated values (.CSV) text format files.
DIA method produces a large volume of mass spectra from the narrow isolation windows it acquires during MS analysis. Spectronaut (https://biognosys.com/ software/spectronaut/) is a good software for analyzing large experiment DIA MS datasets [7]. Spectronaut is commercially licensed and requires the iRT Kit as a protein standard for calibration and quality control. The spectral library is a prerequisite to performing runs in Spectronaut for deep proteomic profiling of thousands of proteins in a single analysis. The spectral library is generated in Spectronaut using the MS data raw files from digested proteins of different samples. With similar parameters and conditions in the same instrument used for MS runs, several to hundreds of MS raw data can be processed to produce a very large spectral library. For a more comprehensive investigation of the breast cancer proteome, sample pre-fractionation steps improve its outcomes [12, 13].
The mass of these precursor ions detected passes stringent criteria, q-value, and FDR cutoffs before being reported in the final summary of results. MS raw data from the samples and the iRT standards are initially processed in either Maxquant, Mascot, or other search engines like Protein Pilot and Proteome Discoverer. Search files in their original formats, i.e., Maxquant as msms.txt or evidence.txt, or from Mascot in *.dat, and the peptide modifications used are submitted to Spectronaut for the generation of the spectral library. Spectronaut will perform the assay using a combination of MS internal modification databases and external search engines to produce a deep and comprehensive spectral library. Another option in Spectronaut is to import spectral libraries and merge them with new or existing libraries. Although possible, this action is not highly recommended due to the software’s recalibration, resulting in an uncontrolled adjustment of the protein FDR causing the loss of a few hundred proteins in identification. The spectral library workflow can successfully produce a breast cancer tissue-spectral library with a large number of proteome data [14]. Spectronaut performance in generating either the spectral library or actual proteome sample analysis is calibrated with iRT peptide standards at 1% FDR. The generated spectral library results from stringent DIA criteria to analyze convoluted MS datasets of tissue samples. Like Mascot and Maxquant, Spectronaut performs peptide matching using the peptide-centric method by performing peptide queries against its MS/MS spectra database. The results obtained are comprehensive visualizations and quantifiable information about the analysis, the MS data acquisition, proteome abundance, and post-analysis results.
Unlike Spectronaut, MS raw data search engines are freely available online for non-profit and academic use. These search engines also perform the general MS raw data processing, quantitation, and additional biological annotations. These tools include Spectrum Mill MS (https://proteomics.broadinstitute.org/millhome. htm), Proteome Discoverer (https://bit.ly/3AqbGmv), PEAKS (https://www.bioinfor.com/peaks-online/), X!! Tandem (https://wiki.thegpm.org/wiki/X!!Tandem), and ProteinPilot (https://sciex.com/products/software/ proteinpilot-software), among others.
With the development and continuous improvement in sophisticated software and user-friendly interface applications for proteomic analysis, a historical leap in protein biomarker discovery makes it evident to confidently arrive at a vast array of proteome information with fewer false positives and missing data. Depending on which software is used for the analysis, a tabulated summary of results will be the final goal in the search analysis. In general, these tools give a summarized report on the aligned sequences from the sequence similarity search, database ID, source of the sequence, precursor, peptide and protein sequences, number of matching peptides, mass abundance or intensities, gene ontologies, length of the proteome sequence, score, p-value, query number for MS/MS spectra details of the ion, the theoretical mass of the closest-matching peptide, and the observed theoretical difference, the experimental mass/ charge of the ion, and the calculated uncharged mass, to name a few of the most common information we find in the summary table, maybe more or less depending on the software used. Some software like Spectronaut also gives visualization on the precursor, peptide, protein ratio, histogram, heatmaps, volcano plot, and box plots. These are useful for closely investigating differentially expressed protein, relative protein expression, protein-protein interactions, and protein post-modifications. These are the most sought-after and interesting data we can collect from the analysis to best understand molecules involved in the complex biology of breast cancer.
Proteomics Bioinformatics Tools
The MS spectra and the summary of results can now be further subjected to evaluation, functional annotation, and biochemical pathways analysis. The Swiss Institute of Bioinformatics (SIB, https://www.sib.swiss/) is one of the pioneers in developing quality online bioinformatics tools for proteomics and other omics applications. Among the tools they developed are UniProtKB, STRINGDb, and various Expasy’s (https://www.expasy. org/search/proteins%20proteomes/) tools designed for mapping proteins in complex biochemical pathways, ontologies and networks, and other omics applications. The UniProt Knowledgebase (UniProtKB, https://www. uniprot.org/) is composed of UniProt/Swiss-Prot and UniProtKB/TrEMBL. UniProt/Swiss-Prot is created from experimentally proven scientific literature results and curator-reviewed computational analysis producing a high- quality database of manually annotated and non-redundant protein sequences and functional information.
In contrast, UniProtKB/TrEMBL are automatically annotated online without undergoing a review. UniProtKB and its newer beta version give a quick overview of protein information and citations. The search summary includes the amino acid sequence, protein name and description, protein structure, gene name, taxonomic data, pathways, genes, other biological ontologies, related diseases, and cross-references. STRINGDb (https://string-db.org/) is a versatile database used for network functional enrichment analysis of proteins and protein-protein interactions (PPI). Biological databases and up-to-date web-based resources are foundations for these essential interactions. The US Food and Drug Administration (US FDA) has listed approved clinical biomarkers for breast cancer [15]. Figure 2 shows the PPI of clinically approved breast cancer biomarkers - estrogen receptor 1 (ESR1), breast cancer type 1 susceptibility protein (BRCA1), breast cancer type 2 susceptibility protein (BRCA2), and receptor tyrosine- protein kinase erbB-2 (ERBB2, also known as HER2).
Figure 2. Protein Network of Known Clinical Breast Cancer Biomarkers – BRCA1, BRCA2, ESR1 and ERBB2.
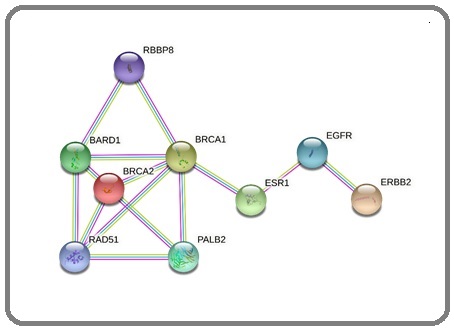
Demonstrating the use of STRINGDb online, we have observed strong evidence that associates these biomarkers with one another from validated and peer-reviewed experiments and current databases. Interestingly we discovered the other interactors, namely BRCA1-associated ring domain protein 1 (BARD1) and partner and localizer of BRCA2 (PALB2). These genes have been previously linked to the prevalence of breast cancer [16-18]. StringDB also presents a summary of information, an interactive Protein Data Bank (PDB) structures (https://www.rcsb.org/) and homology models about each node, and links to explore more protein and gene data resources. The National Center for Biotechnology Information (NCBI) (https://www.ncbi. nlm.nih.gov/), GenBank (https://www.ncbi.nlm.nih.gov/ genbank/) [19], NextProt (https://www.nextprot.org/) [20], Ensembl (https://oct2014.archive.ensembl.org/), and Simple Modular Architecture Research Tool (SMART) (https://smart.embl.de/) (18) among others are quick links presented for every protein shown in the StringDB PPI network (Figure 2).
Significantly, NCBI has always been the most reliable source of the latest public health and research information for the life sciences, including protein data. NCBI offers an interactive online platform that allows users to download data manuscripts for research, learn from the integrated tutorials, use bioinformatics tools and their applications, and perform diverse task analyses. NCBI has a section particular for proteins, namely the Protein Database and the Reference Sequence Database (RefSeq), including genomic, transcript, and protein from more than 115,000 organisms, the Third-Party Annotation (TPA) Sequence, and the Basic Local Alignment Search Tool (BLAST). In addition to the many online resources, the Human
Protein Atlas portal (http://www.proteinatlas.org/) has complete information about the human proteins of the different parts of the body, normal and cancer tissues, blood, single cell, tissue cells, immune cells, and cell lines [21]. It has combined various omics technologies, systems biology, and bioinformatics to create open-source access to spatial human proteome data. The sample preparation and treatment can be performed in different areas such as transcriptional, post-transcriptional, translation, promoter analysis, protein expression, and post-translation modification. The information is organized systematically into other aspects of the study on the genome-wide analysis of the human proteome. Genes are considered most important in HPA, and it includes metabolic genes related to hundreds of metabolic pathways and metabolic processes involving proteins. HPA also consists of a dedicated section for pathology, which contains protein and mRNA expression from different cancers. Pathology reports also include the immunohistochemistry images of the stained cancer tissues.
While we are primarily focused on studying proteins, efficiently mapping the coding genes for each protein will accelerate our search for an insightful interpretation of our candidate biomarkers in their effects on biochemical pathways and molecular systems. The tools we often use are Kyoto Encyclopedia of Genes and Genomes (KEGG), GeneCards, Protein Analysis Through Evolutionary Relationships (PANTHER), Database for Annotation, Visualization, and Integrated Discovery (DAVID), BioRender, cBioPortal, and Ingenuity Pathway Analysis (IPA).
KEGG (https://www.genome.jp/kegg/) mapper in KEGG is an online bioinformatics tool for mapping the protein candidates’ coding genes in related breast cancer biochemical pathways [22]. We used the color tool in the KEGG mapper to quickly find the coding genes for BRCA1, BRCA2, ESR1, ERBB2, BARD1, and PALB2.
These UniProt gene IDs are first converted to KEGG IDs before executing the search and color application. The analysis showed 4 out of the 6 coding genes of the protein of interest in the breast cancer pathway. From this illustration (Figure 3), the breast cancer signaling pathway shows the expression of ESR1, BRCA1, BRCA2, and ERBB2.
Figure 3. Coding Genes of ESR1, ERBB2, BRCA1, and BRCA2 Proteins are Shown in the Purple Shade in the Breast Cancer Pathway Searched in the KEGG Mapper Tool.
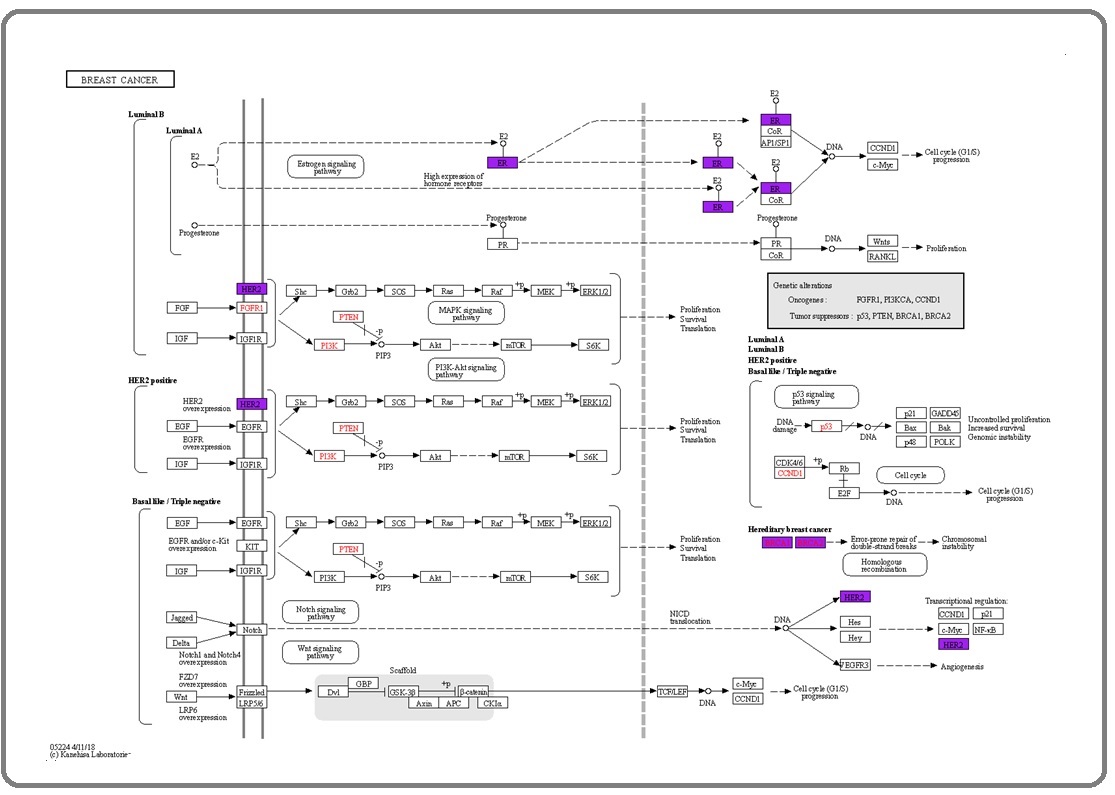
The most common breast cancer shows an uncontrolled expression of ESR1. ESR1 is a known prognostic biomarker linked indirectly to breast cancer progression by binding the hormone estrogen to the estrogen receptor). The activation of the estrogen signaling pathway cascades signal that eventually activates phosphoinositide-3- kinase (PI3K) and mitogen-activated protein kinase (MAPK) signaling pathways which promote breast cancer cells to proliferate and become more invasive [23, 24]. HER2-positive breast cancer is supported by the ERBB2 dimerization and amplification on the cell membrane. As a result, the dimer would activate a cascade of signals affecting MAPK and P13K signaling pathways [25]. If HER2 is overexpressed, downstream activation of the molecules follows, resulting in breast cancer cell proliferation, survival, and translation [26]. Some patients with familial history of breast cancer are diagnosed with biomarkers BRCA1 and BRCA2 before its onset. The BRCA1 and BRCA2 gene mutations are associated with hereditary DNA damage response, leading to an increased risk of developing breast cancer [27,28]. The most effective medical practice for BRCA1 or BRCA2 carriers is to have their breast removed to prevent breast cancer. However, the prevalence of breast cancer and the presentation of the gene mutation among women have been different across different ethnicities [29]. Figure 3 shows that the other genes, PALB2 and BARD1, did not appear in the canonical pathway; however, some studies have already associated that mutation of these genes increases the risk of breast cancer [30,31]. BARD1 was reported to form a heterodimer complex with BRCA1 enhancing its ubiquitin ligase activity, and is suspected to affect DNA repair [16].
GeneCards (https://www.genecards.org/), in addition to the above, is a convenient source of gene information derived from an integration of multi-omics, genetics, and clinical studies [32]. GeneCards has been the most extensive home page for exploring molecular features, 2D/3D protein structures, gene and protein functions, expression, PPIs, current drugs, ongoing drug clinical trials, and easy links to let you jump from one section to another. It also has a search bar for an easy keyword-based literature search on protein or gene of interest.
PANTHER, like KEGG, is a bioinformatics tool designed to analyze genes, transcripts, and classify proteins according to family, molecular functions, biological processes, and Reactome pathways (https:// reactome.org/). PANTHER allows us to easily include and exclude information we wish to extract from its database into a customizable text file showing the summary of results. In addition, an interactive protein functions analysis displays the results in bar or pie charts. We subjected the 6 proteins - BRCA1, BRCA2, ESR1, ERBB2, BARD1, and PALB2 to PANTHER database analysis, and Figure 4 shows that 4 out of 6 breast cancer proteins are involved in different biological processes. ERBB2 is associated with signaling, developmental, and multicellular organismal processes. BRCA1, BRCA2, and ERBB2 are related to the biological regulation process in the cell.
Figure 4. Panther Pathway Analysis Mapped 2 Pathways (1 out of 6 proteins). ERBB2 is found to interact in both cadherin and EGF receptor signaling pathways.
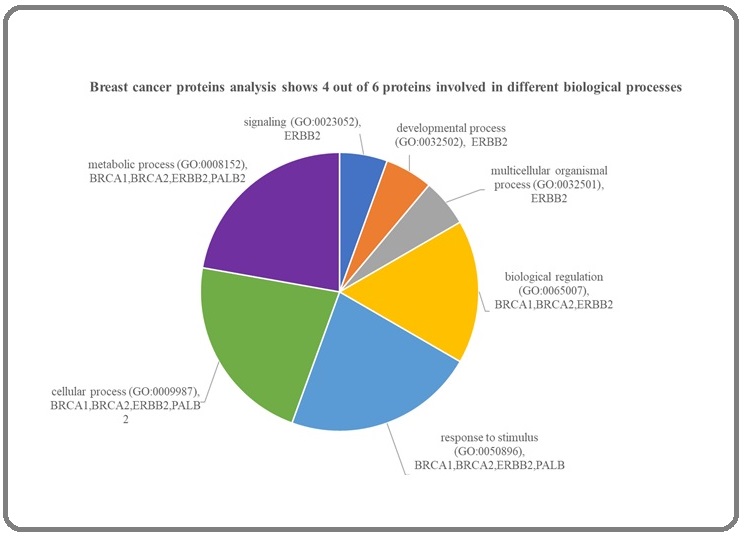
BRCA1, BRCA2, ERBB2, and PALB2 are involved in response to stimulus and various cellular and metabolic processes. PANTHER did not map ESR1 and BARD1, but UniProt shows that ESR1 [33] protein is involved in transcription and regulation, while BARD1 is associated with cancer-related genes involved in damaged DNA repair [34].
DAVID (https://david.ncifcrf.gov/home.jsp) is another excellent high-throughput functional and sequence annotation tool providing a vast array of information to explore genes, gene products, and interacting proteins. In this online tool, users can search up to a maximum of 3000 gene IDs, including clustering and visualization of the big data. DAVID database is created from publicly available resources with clickable links to ontologies, protein domains, pathways, protein interactions, diseases, and protein expression. At this point, it is clear to say that there are many options for us to use when annotations and ontologies are our concern. In addition, the cBioPortal (https://www.cbioportal.org/) is a multinational team designed open-access resource primarily for cancer genomic and proteomic related studies. The portal enables us to observe the coding genes of our proteins in multiple cancer genome databases and cancer research cohort studies. We queried for the coding genes of the 6 proteins in the cBioPortal breast cancer online database and observed them in a combined investigation with 35,912 breast cancer patient samples. The cohort reported that 6888 (2%) patients were found to have alterations in the queried 6 genes in the comparative analysis. Among the genes, the oncoprint tab reports that ERBB2 shows the highest alteration at 9% (3044/35912), followed by BRCA2 (5%), ESR1 (4%), BRCA1 (2.8%, PALB2 (2.1%), and BARD1 (1.2%). Mostly the genes have alterations that are either amplified or missense among the patients, which may increase the probability of mutations leading to cancer development and growth. Patients carrying BRCA2 alterations have a higher chance of manifesting PALB2 alterations than those carrying BRCA1 alterations [35].
Finally, proteomics experiments generate extremely large volumes of raw datasets. For this reason, experimental results can be submitted to an online public proteomic repository for general utilization. The proteomic repository became a systematic and centralized way of storing data that can be made publicly available for scientific information and use. Proteome Xchange [36] [37] (http://www.proteomexchange.org/), SWATHAtlas [38] (http://www.swathatlas.org/), Peptide Atlas [39] (http://www.peptideatlas.org/), MassIVE (https://massive. ucsd.edu/ProteoSAFe/static/massive.jsp), SRMAtlas (http://www.srmatlas.org/), NeXtProt (https://www. nextprot.org/), PRIDE (https://www.ebi.ac.uk/pride/) are some of the many popular and reliable repositories for proteome only with their supporting pieces of evidence from annotated and reviewed literature. The repositories also allow interactive and real-time browsing, analysis, and free downloading. These tools make it easy to access global mass spectrometry data generated using a wide array of MS technologies. Through the years, it has accepted and stored an extensive proteomic profile from diverse species all over the globe. The advanced data analysis algorithms integrated with these user-friendly interfaces make it easy for scientists and researchers to reutilize these datasets to create more opportunities for progress and collaboration in the scientific community, leading to significant discoveries. In conclusion, The established role and importance of proteins in breast cancer research are so compelling that many experts are now utilizing genomics and proteomics in their approach to novel biomarker discovery. Proteomics is even more powerful with the state-of-the-art modern technology and computing systems integration offered by mass spectrometry. Big data generated from MS experiments is also not a challenge because of the availability of diverse and advanced bioinformatics tools and up-to-date databases to analyze the data. This creates an opportunity for the scientific community to fast track their research progress and discover candidate protein molecules for potential clinical trials.
References
- Breast Cancer. Retrieved 18January2022, from https://www.who.int/news-room/fact-sheets/detail/breast-cancer WHO . 2021.
- Philippines has highest prevalence of breast cancer among 197 countries - ManilaMed REFERENCE DW 2017. The Manila Times Vardeleon M. 2017.
- Mass Spectrometry: Principles and Applications (Third Edit) Edmond de Hoffman , Vincent Stroobant . John Wiley & Sons.2007.
- Rapid identification of proteins by peptide-mass fingerprinting Pappin D. J. C., Hojrup P., Bleasby A. J.. Current Biology.1993;3(6). CrossRef
- Chemistry, Mass Spectrometry and Peptide-Mass Databases: Evolution of Methods for the Rapid Identification and Mapping of Cellular Proteins Pappin, Darryl J, Rahman D, Hansen, H. F , Bartlet-Jones, M, Jeffery, W. A , &Bleasby, A. J . 1996.
- Andromeda: a peptide search engine integrated into the MaxQuant environment Cox J, Neuhauser N, Michalski A, Scheltema RA , Olsen JV , Mann M. Journal of Proteome Research.2011;10(4). CrossRef
- Spectronaut: a fast and efficient algorithm for MRM-like processing of data independent acquisition (SWATH-MS) data Bernhardt O, Selevsek N, Gillet L, Rinner O, Picotti P, Aebersold R, Reiter L. 2014.
- Data-independent acquisition-based SWATH-MS for quantitative proteomics: a tutorial Ludwig C, Gillet L, Rosenberger G, Amon S, Collins, B. C , Aebersold R. Molecular Systems Biology.2018;14(8). CrossRef
- The SWISS-PROT protein sequence database and its supplement TrEMBL in 2000 Bairoch A, Apweiler R. Nucleic Acids Research.2000;28(1). CrossRef
- Visualization of LC-MS/MS proteomics data in MaxQuant Tyanova S, Temu T, Carlson A, Sinitcyn P, Mann M, Cox J. PROTEOMICS.2015;15(8). CrossRef
- MaxQuant enables high peptide identification rates, individualized p.p.b.-range mass accuracies and proteome-wide protein quantification Cox J, Mann M. Nature Biotechnology.2008;26(12). CrossRef
- Rapid High-pH Reverse Phase StageTip for Sensitive Small-Scale Membrane Proteomic Profiling Dimayacyac-Esleta BRT , Tsai C, Kitata RB , Lin P, Choong W, Lin T, Wang Y, Weng S, Yang P, Arco SD , Sung T, Chen Y. Analytical Chemistry.2015;87(24). CrossRef
- Mining Missing Membrane Proteins by High-pH Reverse-Phase StageTip Fractionation and Multiple Reaction Monitoring Mass Spectrometry Kitata RB , Dimayacyac-Esleta BRT , Choong W, Tsai C, Lin T, Tsou C, Weng S, Chen Y, Yang P, Arco SD , Nesvizhskii AI , Sung T, Chen Y. Journal of Proteome Research.2015;14(9). CrossRef
- High-precision iRT prediction in the targeted analysis of data-independent acquisition and its impact on identification and quantitation Bruderer R, Bernhardt OM , Gandhi T, Reiter L. Proteomics.2016;16(15-16). CrossRef
- Breast cancer drug approvals by the US FDA from 1949 to 2018 Leo CP , Leo C, Szucs TD . Nature Reviews. Drug Discovery.2020;19(1). CrossRef
- Isomerization of BRCA1-BARD1 promotes replication fork protection Daza-Martin M, Starowicz K, Jamshad M, Tye S, Ronson GE , MacKay HL , Chauhan AS , Walker AK , Stone HR , Beesley JFJ , Coles JL , Garvin AJ , Stewart GS , McCorvie TJ , Zhang X, Densham RM , Morris JR . Nature.2019;571(7766). CrossRef
- Prevalence of disease-causing genes in Japanese patients with BRCA1/2-wildtype hereditary breast and ovarian cancer syndrome Kaneyasu T, Mori S, Yamauchi H, Ohsumi S, Ohno S, Aoki D, Baba S, Kawano J, Miki Y, Matsumoto N, Nagasaki M, Yoshida R, Akashi-Tanaka S, Iwase T, Kitagawa D, Masuda K, Hirasawa A, Arai M, Takei J, Ide Y, Gotoh O, Yaguchi N, Nishi M, Kaneko K, Matsuyama Y, Okawa M, Suzuki M, Nezu A, Yokoyama S, Amino S, Inuzuka M, Noda T, Nakamura S. NPJ breast cancer.2020;6. CrossRef
- Identification of a novel pathogenic variant in PALB2 and BARD1 genes by a multigene sequencing panel in triple negative breast cancer in Morocco Laraqui A, Cavaillé M, Uhrhammer N, ElBiad O, Bidet Y, El Rhaffouli H, El Anaz H, Rahali DM , Kouach J, Guelzim K, Badaoui B, AlBouzidi A, Oukabli M, Tanz R, Sbitti Y, Ichou M, Ennibi K, Sekhsokh Y, Bignon Y. Journal of Genomics.2021;9. CrossRef
- GenBank Benson DA , Cavanaugh M, Clark K, Karsch-Mizrachi I, Lipman DJ , Ostell J, Sayers EW . Nucleic Acids Research.2013;41(Database issue). CrossRef
- The neXtProt knowledgebase in 2020: data, tools and usability improvements Zahn-Zabal M, Michel P, Gateau A, Nikitin F, Schaeffer M, Audot E, Gaudet P, Duek PD , Teixeira D, Rech de Laval V, Samarasinghe K, Bairoch A, Lane L. Nucleic Acids Research.2020;48(D1). CrossRef
- Proteomics. Tissue-based map of the human proteome Uhlén M, Fagerberg L, Hallström BM , Lindskog C, Oksvold P, Mardinoglu A, Sivertsson Å, Kampf C, Sjöstedt E, Asplund A, Olsson I, Edlund K, Lundberg E, Navani S, Szigyarto CA C, Odeberg J, Djureinovic D, Takanen JO , Hober S, Alm T, Edqvist P, Berling H, Tegel H, Mulder J, Rockberg J, Nilsson P, Schwenk JM , Hamsten M, Feilitzen K, Forsberg M, Persson L, Johansson F, Zwahlen M, Heijne G, Nielsen J, Pontén F. Science (New York, N.Y.).2015;347(6220). CrossRef
- KEGG: Kyoto Encyclopedia of Genes and Genomes Kanehisa M, Goto S. Nucleic Acids Research.2000;28(1). CrossRef
- Oncogenic PIK3CA-driven mammary tumors frequently recur via PI3K pathway-dependent and PI3K pathway-independent mechanisms Liu P, Cheng H, Santiago S, Raeder M, Zhang F, Isabella A, Yang J, Semaan DJ , Chen C, Fox EA , Gray NS , Monahan J, Schlegel R, Beroukhim R, Mills GB , Zhao JJ . Nature Medicine.2011;17(9). CrossRef
- Mitogen-Activated Protein Kinase (MAPK): New Insights in Breast Cancer Lu H, Guo Y, Gupta G, Tian X. Journal of Environmental Pathology, Toxicology and Oncology: Official Organ of the International Society for Environmental Toxicology and Cancer.2019;38(1). CrossRef
- The PI3K/AKT/mTOR and CDK4/6 Pathways in Endocrine Resistant HR+/HER2- Metastatic Breast Cancer: Biological Mechanisms and New Treatments Presti D, Quaquarini E. Cancers.2019;11(9). CrossRef
- Treatment of HER2-positive breast cancer Figueroa-Magalhães MC , Jelovac D, Connolly R, Wolff AC . Breast (Edinburgh, Scotland).2014;23(2). CrossRef
- BRCA1 and BRCA2 mutations in women of different ethnicities undergoing testing for hereditary breast-ovarian cancer Hall MJ , Reid JE , Burbidge LA , Pruss D, Deffenbaugh AM , Frye C, Wenstrup RJ , Ward BE , Scholl TA , Noll WW . Cancer.2009;115(10). CrossRef
- Identification of the breast cancer susceptibility gene BRCA2 Wooster R., Bignell G., Lancaster J., Swift S., Seal S., Mangion J., Collins N., Gregory S., Gumbs C., Micklem G.. Nature.1995;378(6559). CrossRef
- BRCA1 and BRCA2 mutations in women of different ethnicities undergoing testing for hereditary breast-ovarian cancer Hall MJ , Reid JE , Burbidge LA , Pruss D, Deffenbaugh AM , Frye C, Wenstrup RJ , Ward BE , Scholl TA , Noll WW . Cancer.2009;115(10). CrossRef
- Breast-cancer risk in families with mutations in PALB2 Antoniou AC , Casadei S, Heikkinen T, Barrowdale D, Pylkäs K, Roberts J, Lee A, Subramanian D, De Leeneer K, Fostira F, Tomiak E, Neuhausen SL , Teo ZL , Khan S, Aittomäki K, Moilanen JS , Turnbull C, Seal S, Mannermaa A, Kallioniemi A, Lindeman GJ , Buys SS , Andrulis IL , Radice P, Tondini C, Manoukian S, Toland AE , Miron P, Weitzel JN , Domchek SM , Poppe B, Claes KBM , Yannoukakos D, Concannon P, Bernstein JL , James PA , Easton DF , Goldgar DE , Hopper JL , Rahman N, Peterlongo P, Nevanlinna H, King M, Couch FJ , Southey MC , Winqvist R, Foulkes WD , Tischkowitz M. The New England Journal of Medicine.2014;371(6). CrossRef
- Triple-Negative Breast Cancer Risk Genes Identified by Multigene Hereditary Cancer Panel Testing Shimelis H, LaDuca H, Hu C, Hart SN , Na J, Thomas A, Akinhanmi M, Moore RM , Brauch H, Cox A, Eccles DM , Ewart-Toland A, Fasching PA , Fostira F, Garber J, Godwin AK , Konstantopoulou I, Nevanlinna H, Sharma P, Yannoukakos D, Yao S, Feng B, Tippin Davis B, Lilyquist J, Pesaran T, Goldgar DE , Polley EC , Dolinsky JS , Couch FJ . Journal of the National Cancer Institute.2018;110(8). CrossRef
- The GeneCards Suite BT - Practical Guide to Life Science Databases (I.Abugessaisa &T.Kasukawa, Eds.). https://doi.org/10.1007/978-981-16-5812-9_2 Safran M, Rosen N, Twik M, BarShir R, Stein, T. I. , Dahary D, Lancet D. 2021.
- Estrogen receptor (ER)-mediated transcriptional regulation of the human corticotropin-releasing hormone-binding protein promoter: differential effects of ERalpha and ERbeta Stolpe A, Slycke AJ , Reinders MO , Zomer AWM , Goodenough S, Behl C, Seasholtz AF , Saag PT . Molecular Endocrinology (Baltimore, Md.).2004;18(12). CrossRef
- BRCA1/BARD1 inhibition of mRNA 3' processing involves targeted degradation of RNA polymerase II Kleiman FE , Wu-Baer F, Fonseca D, Kaneko S, Baer R, Manley JL . Genes & Development.2005;19(10). CrossRef
- PALB2 (partner and localizer of BRCA2) Hanenberg H, Andreassen PR . Atlas of Genetics and Cytogenetics in Oncology and Haematology.2018;22(12). CrossRef
- The ProteomeXchange consortium in 2017: supporting the cultural change in proteomics public data deposition Deutsch EW , Csordas A, Sun Z, Jarnuczak A, Perez-Riverol Y, Ternent T, Campbell DS , Bernal-Llinares M, Okuda S, Kawano S, Moritz RL , Carver JJ , Wang M, Ishihama Y, Bandeira N, Hermjakob H, Vizcaíno JA . Nucleic Acids Research.2017;45(D1). CrossRef
- ProteomeXchange provides globally coordinated proteomics data submission and dissemination Vizcaíno JA , Deutsch EW , Wang R, Csordas A, Reisinger F, Ríos D, Dianes JA , Sun Z, Farrah T, Bandeira N, Binz P, Xenarios I, Eisenacher M, Mayer G, Gatto L, Campos A, Chalkley RJ , Kraus H, Albar JP J, Martinez-Bartolomé S, Apweiler R, Omenn GS , Martens L, Jones AR , Hermjakob H. Nature Biotechnology.2014;32(3). CrossRef
- Retrieved from 2022 website: swathatlas.org SWATHAtlas. (n.d.) . .
- The PeptideAtlas project Desiere F, Deutsch EW , King NL , Nesvizhskii AI , Mallick P, Eng J, Chen S, Eddes J, Loevenich SN , Aebersold R. Nucleic Acids Research.2006;34(Database issue). CrossRef
License

This work is licensed under a Creative Commons Attribution-NonCommercial 4.0 International License.
Copyright
© Asian Pacific Journal of Cancer Biology , 2022
Author Details